Talk:Gamma distribution
![]() | This article is rated B-class on Wikipedia's content assessment scale. It is of interest to the following WikiProjects: | ||||||||||||||||||||
|
Archives: 1 |
|
This page has archives. Sections older than 30 days may be automatically archived by Lowercase sigmabot III when more than 5 sections are present. |
Median of the gamma distribution
References that seems like self promotion
I'm not sure about the pertinence of reference 5, it seems really just plugged from a random journal 'just because' rather than being useful. This is the reference Banneheka BMSG, Ekanayake GEMUPD (2009) "A new point estimator for the median of gamma distribution". Viyodaya J Science, 14:95–103 I'd suggest just removing that passage really. — Preceding unsigned comment added by 143.121.239.188 (talk) 14:27, 24 July 2019 (UTC)
- It looks like a good accessible reference for an excellent approximate formula for the median. Why do you see it as problematic? Dicklyon (talk) 15:40, 24 July 2019 (UTC)
- Does it? It really just look like a low level effort in a random journal after reading it. Kind of fell cheated because it is just a simple linear regression over the values, nothing special. I was expecting a real reason/formula with a theoretical justification in fact, it looks more like on the level of a blog post rather than a rigorous mathematical development. -> Math bachelor here, now finishing PhD in science (and same guy as above). — Preceding unsigned comment added by 145.130.146.105 (talk) 20:57, 24 July 2019 (UTC)
- Well, they note it's a simple approximation to a function with no simple closed form; at least they analyze the accuracy. You suggested self promotion; did you find that it was posted here by its author(s)? Dicklyon (talk) 21:16, 24 July 2019 (UTC)
- Does it? It really just look like a low level effort in a random journal after reading it. Kind of fell cheated because it is just a simple linear regression over the values, nothing special. I was expecting a real reason/formula with a theoretical justification in fact, it looks more like on the level of a blog post rather than a rigorous mathematical development. -> Math bachelor here, now finishing PhD in science (and same guy as above). — Preceding unsigned comment added by 145.130.146.105 (talk) 20:57, 24 July 2019 (UTC)
- No, I just expected reference in wikipedia to be textbooks or established references in the field rather than anecdotic evidence at best. I also have been fixing other pages which were blatantly wrong/now much better understood in my field (that's the nice thing with this, everyone can write stuff, but now I realize the more I know that really anyone writes here). Figured it must be better to have solid evidence for newcomers to this page rather than not certain/not super good approximations. Anyway, it's true I can't suggest better, but it is of limited use (they tested for alpha = 1 to 20 if memory serves, or something limited. The main issue I have is mostly it seems to be from a random journal with a small readership, if any. It's already tedious to sift through the nonsense on internet at large when looking for manuscripts, figured only well curated/known information should make it here only. — Preceding unsigned comment added by 143.121.239.188 (talk • contribs)
- Just FYI, we generally do not cite textbooks. They are tertiary sources, and therefore weak. See WP:USETERTIARY for some analysis. — SMcCandlish ☏ ¢ 😼 08:00, 11 October 2020 (UTC)
- This applies to citing them for analytic, evaluative, interpretive, or synthetic claims. For factual claims, tertiary sources at an academic level are generally fine. --Lambiam 19:34, 7 December 2024 (UTC)
- Just FYI, we generally do not cite textbooks. They are tertiary sources, and therefore weak. See WP:USETERTIARY for some analysis. — SMcCandlish ☏ ¢ 😼 08:00, 11 October 2020 (UTC)
- No, I just expected reference in wikipedia to be textbooks or established references in the field rather than anecdotic evidence at best. I also have been fixing other pages which were blatantly wrong/now much better understood in my field (that's the nice thing with this, everyone can write stuff, but now I realize the more I know that really anyone writes here). Figured it must be better to have solid evidence for newcomers to this page rather than not certain/not super good approximations. Anyway, it's true I can't suggest better, but it is of limited use (they tested for alpha = 1 to 20 if memory serves, or something limited. The main issue I have is mostly it seems to be from a random journal with a small readership, if any. It's already tedious to sift through the nonsense on internet at large when looking for manuscripts, figured only well curated/known information should make it here only. — Preceding unsigned comment added by 143.121.239.188 (talk • contribs)
- To me it looks inferior to the Choi formula. I would recommend simply the first line of this section, "Unlike ... = 1/2" and then
- The asymptotic expansion of the median is, for k > 1 and θ > 0 [1] [2]
- The fact that the median is concave is trivially obvious from this formula by differentiating twice --- it doesn't need a new reference --- I would say: "The median is therefore a concave function of k for constant \theta as can be seen by differentiating this expansion twice."
- — Preceding unsigned comment added by Nick Mulgan (talk • contribs)
References
- ^ Jeesen Chen, Herman Rubin, Bounds for the difference between median and mean of gamma and poisson distributions, Statistics & Probability Letters, Volume 4, Issue 6, October 1986, Pages 281-283, ISSN 0167-7152, [1].
- ^ Choi, K.P. "On the Medians of the Gamma Distributions and an Equation of Ramanujan", Proceedings of the American Mathematical Society, Vol. 121, No. 1 (May, 1994), pp. 245–251.
- If you mean differentiating the Laurent series, no, you can't do that, since it's not convergent near 0. Dicklyon (talk) 04:31, 11 March 2020 (UTC)
- Besides, the next two terms are negative and concave, as shown after Choi, by Berg & Pedersen (see article that I update since your comment here). Dicklyon (talk) 19:08, 17 May 2021 (UTC)
One of the big problems in that section is that the different parts use different terminology. Both could use m, or equivalently the ratio ν/µ of median to mean. The other problem is that the bilinear or rational function approximation could be better; adjusting to match the Laurent series version at 1, for example, might make sense. When I do that, I get:
or
where the 0.178 is still a numerical approximation. Someone better at calculus and algebra can probably find the exact value from the 8/405 coefficient. Then again, the next term has the wrong sign, so it's not better than just truncating the Laurent series after the 8/405 term (especially for m < 1), and isn't really simpler. On the other hand, they represent it as being good only for m > 1, so maybe matching a Laurent series around m = 2 or thereabouts would be good, and maybe their original formula does match at some such point? Too bad; the source is not deep enough to use to try to fix this. So I've changed my mind and agree we should probably just drop it. Dicklyon (talk) 05:43, 5 March 2020 (UTC)
- Agreed about terminology being confusing. The Choi paper has m for the mean and for the median which is very confusing and has been copied over to here. I recommend changing to \mu and "the median, m, ... "Nick Mulgan (talk) 01:09, 6 March 2020 (UTC)
- I agree; please do that. Dicklyon (talk) 22:02, 10 March 2020 (UTC)
Better median approximations
Working on this a bit more, I see that the "improvement" I made to match the Laurent series just made it fit worse, mostly. The Laurent series does better above and near 1, but neither is much good below, where they overshot the known bound of 1 – 1/3. Dicklyon (talk) 04:28, 11 March 2020 (UTC)
So I did a bit more "original research" on this. The Laurent series and the bilinear function both fail badly (diverge) for alpha << 1. The median should stay above 0, and approach the mean at very low alpha, like the Banneheka ref shows, but doesn't address. They provide a linear approximation mean/(mean–median) = 0.2+3*alpha, but they don't limit it to not go below 1. If we do that with a sort of soft max, e.g. (1 + (0.2+3*alpha)^p)^(1/p), we can make their approximation work well all over. By adjusting the 0.2 and the power p, we can make it match both the high-alpha limit of the tight Laurent series and the exact value median/mean = log(2) at alpha = 1. Then we have a function that's good across the whole range, is convex everywhere, and is tight to the known good point at 1 and the limits at 0 and infinity. I can't say more about how good it is, as I haven't tried to evaluate the median in between numerically, but its error is less then 1e-5 at alpha values 0, 1, 10, and 20; not quite as good, but within 1e-4 of the Laurent series at alpha 2 and 5.
-
- where
- with
- where
Any mathematicians/statisticians want to write up such a thing and publish it so we can cite it? Dicklyon (talk) 04:32, 12 March 2020 (UTC)
Actually, I messed it up at 0. I'll need to fix that and re-optimize. Dicklyon (talk) 05:39, 12 March 2020 (UTC)
OK, this is better:
-
- where
- with and
- where
which is better than 2e-5 wherever I check. Dicklyon (talk) 05:51, 12 March 2020 (UTC)
The trouble with the bilinear rational function (3α - 1 + c) / (3α + c) of Banneheka is that it needs c = (3*log(2) - 2) / (1 - log(2)) = 0.25889 to match log(2) at α = 1, and it needs c = 72/409 = 0.17604 to match the Laurent series of Choi as α gets large. Can't have it both ways. But one more parameter p in addition to c makes it work at those points, in this form that's designed to also make it work right at α = 0. Now I see that the best c for very large α still needs to 0.17604, and my 0.1814 was just because I wasn't looking at high enough α (assuming Choi's analysis is correct). The corresponding best p value to match log(2) at α = 1 is p = 2.3767. So this is it, really:
-
- where
- with and
- where
where α could be k depending on what notation one prefers here. Dicklyon (talk) 05:36, 14 March 2020 (UTC)
But wait – I did some numerical integration to get pretty accurate true median values, and there's still a big problem with my approximation that's exact at 0, 1, and infinity. It's median estimate is too high by an absolute amount peaking at about 0.0125 at about alpha = 0.22. And the error relative to the true median gets exponentially bad as alpha goes to zero. E.g. at alpha = 0.1 the true median is 0.000593, but my approximation gives an order of magnitude higher at 0.00589 (times the mean).
This is good though, as it led me to how to compute an accurate low-alpha approximation by doing the integral of the pdf while ignoring the exponential factor, since that factor changes neglibibly up to the median when the median is so small compared to the mean. Thus at low alpha we find:
which at 0.1 gives pretty exactly the right answer 0.000593; and at 0.2 gives 0.0204, where correct is 0.0207 and my previous approximation was 50% too high at 0.0328. Not bad. The exponential factor gets down to about exp(-0.02) = 0.98 near the median in the latter case, but the pdf is heavily weighted to the left of the median, so it makes somewhat less than 2% difference in the estimate. For higher α values, it gets worse; 0.5 instead of log(2) at α = 1; falling to about 3/8 of the true answer for very large α. No divergence, though it's hard to compute as Gamma(alpha) gets too big for double precision, somewhere around alpha = 170.
So now, just need to find a clever way to interpolate between the low-α and high-α cases, for which we have accurate formulae, with a parameter that be adjusted to take it through log(2) at 1. Or maybe find a fudge factor that goes from 1 to about 8/3 to apply here. I'll keep looking.
Let me know if anyone finds publications with related observations. Dicklyon (talk) 19:19, 15 March 2020 (UTC)
That method can work OK, using the low-m approximation and a complicated fudge factor, up to alpha = 1 or so. But I found an alternative related to a suggestion of Ramanujan, as I read in this paper: use an offset in the Laurent series, pushing the radius of convergence down to near 0. So I tried that, and found this series that works great down to a bit below alpha = 0.1:
- where and coefficients are by least-squares fit with a 1/alpha weight on errors, to values at alpha = 0.1:0.025:8.
This has a relative error less than 4e-4 at alpha values down to 0.1, and less than 4e-5 from alpha = 1 and above, with the right asymptote at high alpha (using one term less it's not nearly as good; more might get it down to a bit lower alpha). It has quite a few fitted parameters, but fits well pretty much all over. For 0 < alpha < 0.1, just estimating median = 0 is not bad, or use the approximation if you can compute it. It's a practical good approximation, but probably not of much interest to mathematicians. Dicklyon (talk) 04:13, 19 March 2020 (UTC)
OK, I found this paper, which gets the 184 right where Choi had 144, which turns out to be a typo or an arithmetic error. This paper also has the asymptotic behavior at α = 0, but doesn't really use it to make a good low α approximation. I'll keep at that. Dicklyon (talk) 00:13, 1 April 2020 (UTC)
More better median approximations
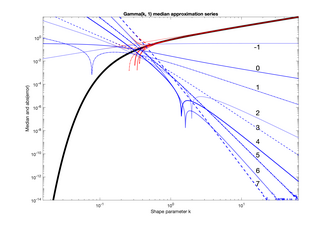
I've written up and illustrated some median approximation formulas on a user subpage: User:Dicklyon/Gamma median, starting with this one that's published and described in the article, and proceeding with some others that work well at lower values of the shape parameter. In case anyone cares. Maybe I'll try to find a way to publish some of this. Any idea where a non-mathematician non-statistician might be able to get such a thing published? Dicklyon (talk) 04:40, 8 April 2020 (UTC)
- I've come up with a simple-ish closed-form pretty good approximation that works across the whole range of shape parameters, with asymptotic absolute and relative errors going to zero at both ends, and with relative error everywhere less than 0.00271 and absolute error everywhere less than 0.00210:
- And with 4 fitted parameters instead of 2, the max errors are a little bit less. Please check me on this, in case I've messed up the translation from Matlab to LaTeX. Dicklyon (talk) 01:36, 6 May 2020 (UTC)
- Actually, it gets better with a different (arctan based or Gudermannian function) interpolator, yielding max relative error below 0.00132:
- Not bad for 2 fitted parameters! Dicklyon (talk) 05:55, 8 May 2020 (UTC)
Closed-from bounds and approximations to the median
I've done a bit more on my explorations, and wrote up a paper that's now on math arXiv: "Closed-form Tight Bounds and Approximations for the Median of a Gamma Distribution". The main point here is that without any numerical work, closed forms can be derived that give great results. It's also submitted to a journal, so with luck I'll get a peer-reviewed publication that's worth referencing for good median bounds and/or approximations. Comments would be welcome. Dicklyon (talk) 03:00, 11 November 2020 (UTC)
Or not. I can't find a journal that wants to even review it. Dicklyon (talk) 03:56, 19 November 2020 (UTC)
Finally got favorable reviews from PLOS ONE. Looks like it will get published. It includes a simpler closed-form approximation than the one above (as in the arXiv version), with closed-form parameters (no decimal approximations), that is exact at k = 1 and has asymptotically zero absolute and relative error at both low and high k. Also some better tight bounds, but they are presented as conjectures since I don't have analytical proofs of them. Dicklyon (talk) 03:26, 30 April 2021 (UTC)
Updated section

I've updated the section Gamma distribution#Median approximations and bounds to represent the state of knowledge of bounds and asymptotic approximations, as published through early 2021, including a plot that represents the remaining big gap between bounds that my work attempts to fill. Maybe if/when my paper gets published, someone will take a look and decide it worth adding something from it, even though the new bounds are characterized as "conjectures", lacking rigorous proof. Dicklyon (talk) 20:12, 9 May 2021 (UTC)
I copied the figure here so it can be viewed in relation to the new figure in the next section. Dicklyon (talk) 21:47, 13 May 2021 (UTC)
The Lyon 2021 paper on median of the gamma distribution

Is published today [2], along with the complete peer review conversation. I will propose here what I think should be added to the article as an update, including a figure that I'm working on. Of course, as author, I'm biased, so will defer to anyone with a better idea. Note that the paper and its figures are CC BY, so could be used in Wikimedia Commons if someone want to get into more detail. Dicklyon (talk) 21:10, 13 May 2021 (UTC)
Added the figure here, with the caption I propose for the article, after the other median bounds plot in the section Gamma distribution#Median approximations and bounds. Proposed new paragraph for the end of that section is:
- In 2021, Lyon proposed the asymptotic closed-form expression and conjectured closed-form values of that make it a tight upper or lower bound, and showed that interpolations between those conjectured bounds can make even tighter bounds (still only conjectured, not proved) or excellent approximations, including one that is exact at (where ).[1] See the figure where the approximation is not visually distinguishable from the underlying curve from numeric simulation.
References
- ^ Lyon, Richard F. (13 May 2021). "On closed-form tight bounds and approximations for the median of a gamma distribution". PLOS One. Retrieved 13 May 2021.
- I have added a paragraph similar to the one above to the article. Nice work! Ozob (talk) 05:16, 15 May 2021 (UTC)
- Thanks, Ozob! I made a minor correction, but there's another one probably needed. Where you have "Additionally, he conjectured that interpolations between these bounds can provide even tighter bounds or excellent approximations to the median" it sounds like the "excellent approximations" are part of what's conjectured. Maybe "excellent" is just an opinion, but the approximation that's exact at k = 1 has closed-form parameters analytically derived. Approximations don't need proofs, so aren't conjectures. But it's hard to break up (unless you use the parenthetical disclaimer as I did above).
- Also, what's your opinion on adding this figure after the other? Dicklyon (talk) 23:00, 15 May 2021 (UTC)
- And thanks for the term "asymptotically tight". It's not from the source, but I wish I had known it so could have used it. My concept of tight was a little different, essentially being in parameter space; being asymptotically tight is too easy (even the bound k is that, right?). Dicklyon (talk) 23:06, 15 May 2021 (UTC)

- Actually, looking around, now I'm not sure what it means; it appears that in big-O notation it just means within a constant factor. Tight is supposed to mean zero margin, or equality, at some point, but for my bounds that point is only in the limit of k going to 0 or infinity. The interpolations have the further nice property that both the absolute and relative margins (or errors) go to zero at both ends of the k domain, whether they are bounds or not, which is one reason they make nice approximations. This much I proved (or showed analytically, if not via formal theorems and proofs). Dicklyon (talk) 04:21, 16 May 2021 (UTC)
- If nobody pipes up soon with a better idea, I'll go ahead and work on fixing the details, and will add the figure.
- Other possible directions would be to also include the two new all-k tight lower bounds that I did prove (almost trivially): (approaching equality in the limit of k = 0) and , (with equality at k = 1) with (neither of which is "closed-form" due to the non-elementary gamma function and exponential integral Ei). Dicklyon (talk) 19:25, 17 May 2021 (UTC)
- Another good thing might be a loglog version of such plots, in which the improvement at low k is more dramatic. I'll work on plots. I'm generally working on alternatives to the plots in my paper. Dicklyon (talk) 01:53, 19 May 2021 (UTC)
- I made and uploaded this plot. Dicklyon (talk) 02:27, 19 May 2021 (UTC)
Statement | The median of a gamma(k, 1) distribution is tightly bounded between functions of the form for values including with lower bound and upper bound . |
---|---|
Symbolic statement | |
Conjectured by | Richard F. Lyon |
Conjectured in | May 2021 |
I see there's a new "mathematical statement" infobox. Here's what mine might look like.
Lacking any pushback or better ideas, I went ahead and added this log-log plot and a few more bound details. Obviously I have a little COI here, so if anyone objects, please tweak, fix, remove, or let me know. Dicklyon (talk) 21:44, 29 May 2021 (UTC)
The Lyon 2023 proofs
I've got a tentative acceptance on a new paper that proves some of the above bounds rigorously. It will be a while before I fix the nits and get it published. Dicklyon (talk) 22:27, 30 May 2023 (UTC)
The paper finally came out today. Some conjectures are proved, with peer review. Dicklyon (talk) 06:10, 9 September 2023 (UTC)
Is anyone up for updating the article based on this? Or should I do so myself? Dicklyon (talk) 20:56, 10 September 2023 (UTC)
I tweaked the article accordingly. Dicklyon (talk) 03:55, 2 October 2023 (UTC)
Gamma distribution Method of Moments Estimators
I believe an edit is needed to the Gamma distribution page. In the Method of Moments text box at the top of the page, please add:
Information to be added:
Explanation of issue: If you calculate k and theta using the mean and variance stated, you can get the following method of moments estimators for theta and k.
References supporting change: Solve the system of equations:
mean = k * θ =>
Replace k in terms of theta:
variance = k* => variance = = mean * θ => θ = =>
Then solve mean equation for k replacing θ with variance/mean.
mean= => k = => k = =>
James Tourkistas — Preceding unsigned comment added by Jtourkis (talk • contribs) 22:54, 12 August 2020 (UTC)
- Done, though apparently that template doesn't support a moments2 parameter for an alternate, so I kludged it. Will look further... Dicklyon (talk) 00:57, 13 August 2020 (UTC)
- Fixed now by the good template hacker. Dicklyon (talk) 19:25, 13 August 2020 (UTC)
Notation
In this article, the distribution is referred to variously as
There seems to be no relation as to whether parametrisations are intended with rate or scale. Shouldn't this be harmonized throughout the article? Deviating notation used in other texts should be pointed out in one place, and one notation should be used consistently in this article.
Moreover, it seems to me that in a few other texts, is also used when scale instead of rate is intended, so perhaps one should mention as a caveat that people don't follow these naming conventions strictly.
In particular, Microsoft Excel is very widely used software and it uses a different terminology to that in the article. I suggest adding to the article "Note that Microsoft Excel uses the shape / scale parameterization but calls them alpha and beta." This is very confusing! Source: https://support.microsoft.com/en-gb/office/gamma-dist-function-9b6f1538-d11c-4d5f-8966-21f6a2201def — Preceding unsigned comment added by Blitzer99 (talk • contribs) 15:06, 13 March 2024 (UTC)
- I agree. This issue caused me quite some confusion and wasted time. So I added a note to the page in June 2024. It was removed by somone 2 months later with the comment "removed note on Excel usage; specific product details are not relevant to the general discussion of the distribution". I therfore suggest that we re-introduce this note under a new heading, such as "Notes on software applications" or similar. Here is my proposed note:
- Note about the GAMMA.DIST(,alpha,beta,...) function in Microsoft Excel: The parameters in Microsoft Excel are actually shape and rate parameters respectively, i.e. the correct interpretation of the Microsoft Excel function is GAMMA.DIST(,...). Peter.schild (talk) 15:30, 10 August 2024 (UTC)
- I second this. This caused me quite a headache. I've seen some other places use alpha and beta as shape/scale too, so I'd say that the correct interpretation is GAMMA.DIST(x,shape,scale) to avoid ambiguity. Kmaricq (talk) 03:34, 11 September 2024 (UTC)
CDF
Maybe gammacdf should be 1-F(x) given in the page 201.231.180.105 (talk) 14:13, 5 April 2023 (UTC)
Dark Mode
The first graph on right side looks weird when looking at it in the new dark mode because the font is also dark and doesnt change to white automatically. 147.161.166.247 (talk) 13:27, 6 September 2024 (UTC)
Purposeless complication in the description of parametrizations?
Currently this article says:
Why doesn't it say the following?
Why use two different names, and for the same thing? Michael Hardy (talk) 18:01, 7 December 2024 (UTC)
- And besides, I don't know that I've ever seen the letter k used for this in print. Michael Hardy (talk) 18:41, 7 December 2024 (UTC)
I've changed all the betas to lambdas. That is a far more prevalent usage, and calling the rate parameter beta conflicts with the article titled scale parameter. Michael Hardy (talk) 18:32, 7 December 2024 (UTC)
- . . . and now I've changed all the ks to αs. And noted that in some places the letter k was used as an index of summation, which is another reason why using it as the shape parameter is bad usage. Michael Hardy (talk) 19:29, 7 December 2024 (UTC)
- I agree that your parameter nomenclature changes are more mainstream, but now some plots have ks and the text has αs. --
{{u|Mark viking}} {Talk}
21:26, 7 December 2024 (UTC)
- I agree that your parameter nomenclature changes are more mainstream, but now some plots have ks and the text has αs. --