Swarm behaviour

Swarm behaviour, or swarming, is a collective behaviour exhibited by entities, particularly animals, of similar size which aggregate together, perhaps milling about the same spot or perhaps moving en masse or migrating in some direction. It is a highly interdisciplinary topic.[1]
As a term, swarming is applied particularly to insects, but can also be applied to any other entity or animal that exhibits swarm behaviour. The term flocking or murmuration can refer specifically to swarm behaviour in birds, herding to refer to swarm behaviour in tetrapods, and shoaling or schooling to refer to swarm behaviour in fish. Phytoplankton also gather in huge swarms called blooms, although these organisms are algae and are not self-propelled the way animals are. By extension, the term "swarm" is applied also to inanimate entities which exhibit parallel behaviours, as in a robot swarm, an earthquake swarm, or a swarm of stars.
From a more abstract point of view, swarm behaviour is the collective motion of a large number of self-propelled entities.[2] From the perspective of the mathematical modeller, it is an emergent behaviour arising from simple rules that are followed by individuals and does not involve any central coordination. Swarm behaviour is also studied by active matter physicists as a phenomenon which is not in thermodynamic equilibrium, and as such requires the development of tools beyond those available from the statistical physics of systems in thermodynamic equilibrium. In this regard, swarming has been compared to the mathematics of superfluids, specifically in the context of starling flocks (murmuration).[3]
Swarm behaviour was first simulated on a computer in 1986 with the simulation program boids.[4] This program simulates simple agents (boids) that are allowed to move according to a set of basic rules. The model was originally designed to mimic the flocking behaviour of birds, but it can be applied also to schooling fish and other swarming entities.
Models
In recent decades, scientists have turned to modeling swarm behaviour to gain a deeper understanding of the behaviour.
Mathematical models

External images | |
---|---|
![]() | |
![]() | |
![]() |
Early studies of swarm behaviour employed mathematical models to simulate and understand the behaviour. The simplest mathematical models of animal swarms generally represent individual animals as following three rules:
- Move in the same direction as their neighbours
- Remain close to their neighbours
- Avoid collisions with their neighbours
The boids computer program, created by Craig Reynolds in 1986, simulates swarm behaviour following the above rules.[4] Many subsequent and current models use variations on these rules, often implementing them by means of concentric "zones" around each animal. In the "zone of repulsion", very close to the animal, the focal animal will seek to distance itself from its neighbours to avoid collision. Slightly further away, in the "zone of alignment", the focal animal will seek to align its direction of motion with its neighbours. In the outermost "zone of attraction", which extends as far away from the focal animal as it is able to sense, the focal animal will seek to move towards a neighbour.
The shape of these zones will necessarily be affected by the sensory capabilities of a given animal. For example, the visual field of a bird does not extend behind its body. Fish rely on both vision and on hydrodynamic perceptions relayed through their lateral lines, while Antarctic krill rely both on vision and hydrodynamic signals relayed through antennae.
However recent studies of starling flocks have shown that each bird modifies its position, relative to the six or seven animals directly surrounding it, no matter how close or how far away those animals are.[5] Interactions between flocking starlings are thus based on a topological, rather than a metric, rule. It remains to be seen whether this applies to other animals. Another recent study, based on an analysis of high-speed camera footage of flocks above Rome and assuming minimal behavioural rules, has convincingly simulated a number of aspects of flock behaviour.[6][7][8][9]
Evolutionary models
In order to gain insight into why animals evolve swarming behaviours, scientists have turned to evolutionary models that simulate populations of evolving animals. Typically these studies use a genetic algorithm to simulate evolution over many generations. These studies have investigated a number of hypotheses attempting to explain why animals evolve swarming behaviours, such as the selfish herd theory[10][11][12][13][14] the predator confusion effect,[15][16] the dilution effect,[17][18] and the many eyes theory.[19]
Agents
- Mach, Robert; Schweitzer, Frank (2003). "Multi-Agent Model of Biological Swarming". Advances In Artificial Life. Lecture Notes in Computer Science. Vol. 2801. pp. 810–820. CiteSeerX 10.1.1.87.8022. doi:10.1007/978-3-540-39432-7_87. ISBN 978-3-540-20057-4.
Self-organization

Emergence
The concept of emergence—that the properties and functions found at a hierarchical level are not present and are irrelevant at the lower levels–is often a basic principle behind self-organizing systems.[20] An example of self-organization in biology leading to emergence in the natural world occurs in ant colonies. The queen does not give direct orders and does not tell the ants what to do.[citation needed] Instead, each ant reacts to stimuli in the form of chemical scents from larvae, other ants, intruders, food and buildup of waste, and leaves behind a chemical trail, which, in turn, provides a stimulus to other ants. Here each ant is an autonomous unit that reacts depending only on its local environment and the genetically encoded rules for its variety. Despite the lack of centralized decision making, ant colonies exhibit complex behaviours and have even been able to demonstrate the ability to solve geometric problems. For example, colonies routinely find the maximum distance from all colony entrances to dispose of dead bodies.
Stigmergy
A further key concept in the field of swarm intelligence is stigmergy.[21][22] Stigmergy is a mechanism of indirect coordination between agents or actions. The principle is that the trace left in the environment by an action stimulates the performance of a next action, by the same or a different agent. In that way, subsequent actions tend to reinforce and build on each other, leading to the spontaneous emergence of coherent, apparently systematic activity. Stigmergy is a form of self-organization. It produces complex, seemingly intelligent structures, without need for any planning, control, or even direct communication between the agents. As such it supports efficient collaboration between extremely simple agents, who lack any memory, intelligence or even awareness of each other.[22]
Swarm intelligence
Swarm intelligence is the collective behaviour of decentralized, self-organized systems, natural or artificial. The concept is employed in work on artificial intelligence. The expression was introduced by Gerardo Beni and Jing Wang in 1989, in the context of cellular robotic systems.[23]
Swarm intelligence systems are typically made up of a population of simple agents such as boids interacting locally with one another and with their environment. The agents follow very simple rules, and although there is no centralized control structure dictating how individual agents should behave, local, and to a certain degree random, interactions between such agents lead to the emergence of intelligent global behaviour, unknown to the individual agents.
Swarm intelligence research is multidisciplinary. It can be divided into natural swarm research studying biological systems and artificial swarm research studying human artefacts. There is also a scientific stream attempting to model the swarm systems themselves and understand their underlying mechanisms, and an engineering stream focused on applying the insights developed by the scientific stream to solve practical problems in other areas.[24]
Algorithms
Swarm algorithms follow a Lagrangian approach or an Eulerian approach.[25] The Eulerian approach views the swarm as a field, working with the density of the swarm and deriving mean field properties. It is a hydrodynamic approach, and can be useful for modelling the overall dynamics of large swarms.[26][27][28] However, most models work with the Lagrangian approach, which is an agent-based model following the individual agents (points or particles) that make up the swarm. Individual particle models can follow information on heading and spacing that is lost in the Eulerian approach.[25][29]
Ant colony optimization
External image | |
---|---|
![]() |
Ant colony optimization is a widely used algorithm which was inspired by the behaviours of ants, and has been effective solving discrete optimization problems related to swarming.[31] The algorithm was initially proposed by Marco Dorigo in 1992,[32][33] and has since been diversified to solve a wider class of numerical problems. Species that have multiple queens may have a queen leaving the nest along with some workers to found a colony at a new site, a process akin to swarming in honeybees.[34][35]
- Ants are behaviourally unsophisticated; collectively they perform complex tasks. Ants have highly developed sophisticated sign-based communication.
- Ants communicate using pheromones; trails are laid that can be followed by other ants.
- Routing problem ants drop different pheromones used to compute the "shortest" path from source to destination(s).
- Rauch, EM; Millonas, MM; Chialvo, DR (1995). "Pattern formation and functionality in swarm models". Physics Letters A. 207 (3–4): 185. arXiv:adap-org/9507003. Bibcode:1995PhLA..207..185R. doi:10.1016/0375-9601(95)00624-c. S2CID 120567147.
Self-propelled particles
External videos | |
---|---|
![]() – needs Java |
The concept of self-propelled particles (SPP) was introduced in 1995 by Tamás Vicsek et al.[37] as a special case of the boids model introduced in 1986 by Reynolds.[4] An SPP swarm is modelled by a collection of particles that move with a constant speed and respond to random perturbations by adopting at each time increment the average direction of motion of the other particles in their local neighbourhood.[38]
Simulations demonstrate that a suitable "nearest neighbour rule" eventually results in all the particles swarming together, or moving in the same direction. This emerges, even though there is no centralized coordination, and even though the neighbours for each particle constantly change over time.[37] SPP models predict that swarming animals share certain properties at the group level, regardless of the type of animals in the swarm.[39] Swarming systems give rise to emergent behaviours which occur at many different scales, some of which are both universal and robust. It has become a challenge in theoretical physics to find minimal statistical models that capture these behaviours.[40][41]
Particle swarm optimization
Particle swarm optimization is another algorithm widely used to solve problems related to swarms. It was developed in 1995 by Kennedy and Eberhart and was first aimed at simulating the social behaviour and choreography of bird flocks and fish schools.[42][43] The algorithm was simplified and it was observed to be performing optimization. The system initially seeds a population with random solutions. It then searches in the problem space through successive generations using stochastic optimization to find the best solutions. The solutions it finds are called particles. Each particle stores its position as well as the best solution it has achieved so far. The particle swarm optimizer tracks the best local value obtained so far by any particle in the local neighbourhood. The remaining particles then move through the problem space following the lead of the optimum particles. At each time iteration, the particle swarm optimiser accelerates each particle toward its optimum locations according to simple mathematical rules. Particle swarm optimization has been applied in many areas. It has few parameters to adjust, and a version that works well for a specific applications can also work well with minor modifications across a range of related applications.[44] A book by Kennedy and Eberhart describes some philosophical aspects of particle swarm optimization applications and swarm intelligence.[45] An extensive survey of applications is made by Poli.[46][47]
Altruism
Researchers in Switzerland have developed an algorithm based on Hamilton's rule of kin selection. The algorithm shows how altruism in a swarm of entities can, over time, evolve and result in more effective swarm behaviour.[48][49]
Biological swarming

The earliest evidence of swarm behaviour in animals dates back about 480 million years. Fossils of the trilobite Ampyx priscus have been recently described as clustered in lines along the ocean floor. The animals were all mature adults, and were all facing the same direction as though they had formed a conga line or a peloton. It has been suggested they line up in this manner to migrate, much as spiny lobsters migrate in single-file queues;[50] it has also been suggested that the formation is the precursor for mating,[51] as with the fly Leptoconops torrens. The findings suggest animal collective behaviour has very early evolutionary origins.[52]
Examples of biological swarming are found in bird flocks,[53] fish schools,[54][55] insect swarms,[56] bacteria swarms,[57][58] molds,[59] molecular motors,[60] quadruped herds[61] and people.[62][63][64][65]
Social insects
The behaviour of social insects (insects that live in colonies, such as ants, bees, wasps and termites) has always been a source of fascination for children, naturalists and artists. Individual insects seem to do their own thing without any central control, yet the colony as a whole behaves in a highly coordinated manner.[66] Researchers have found that cooperation at the colony level is largely self-organized. The group coordination that emerges is often just a consequence of the way individuals in the colony interact. These interactions can be remarkably simple, such as one ant merely following the trail left by another ant. Yet put together, the cumulative effect of such behaviours can solve highly complex problems, such as locating the shortest route in a network of possible paths to a food source. The organised behaviour that emerges in this way is sometimes called swarm intelligence, a form of biological emergence.[66]
Ants
Individual ants do not exhibit complex behaviours, yet a colony of ants collectively achieves complex tasks such as constructing nests, taking care of their young, building bridges and foraging for food. A colony of ants can collectively select (i.e. send most workers towards) the best, or closest, food source from several in the vicinity.[67] Such collective decisions are achieved using positive feedback mechanisms. Selection of the best food source is achieved by ants following two simple rules. First, ants which find food return to the nest depositing a pheromone chemical. More pheromone is laid for higher quality food sources.[68] Thus, if two equidistant food sources of different qualities are found simultaneously, the pheromone trail to the better one will be stronger. Ants in the nest follow another simple rule, to favor stronger trails, on average. More ants then follow the stronger trail, so more ants arrive at the high quality food source, and a positive feedback cycle ensures, resulting in a collective decision for the best food source. If there are two paths from the ant nest to a food source, then the colony usually selects the shorter path. This is because the ants that first return to the nest from the food source are more likely to be those that took the shorter path. More ants then retrace the shorter path, reinforcing the pheromone trail.[69]
Army ants, unlike most ant species, do not construct permanent nests; an army ant colony moves almost incessantly over the time it exists, remaining in an essentially perpetual state of swarming. Several lineages have independently evolved the same basic behavioural and ecological syndrome, often referred to as "legionary behaviour", and may be an example of convergent evolution.[70]
The successful techniques used by ant colonies have been studied in computer science and robotics to produce distributed and fault-tolerant systems for solving problems. This area of biomimetics has led to studies of ant locomotion, search engines that make use of "foraging trails", fault-tolerant storage and networking algorithms.[71]
Honey bees

In temperate climates, honey bees usually form swarms in late spring. A swarm typically contains about half the workers together with the old queen, while the new queen stays back with the remaining workers in the original hive. When honey bees emerge from a hive to form a swarm, they may gather on a branch of a tree or on a bush only a few meters from the hive. The bees cluster about the queen and send out 20–50 scouts to find suitable new nest locations. The scouts are the most experienced foragers in the cluster. If a scout finds a suitable location, she returns to the cluster and promotes it by dancing a version of the waggle dance. This dance conveys information about the quality, direction, and distance of the new site. The more excited she is about her findings, the more vigorously she dances. If she can convince others they may take off and check the site she found. If they approve they may promote it as well. In this decision-making process, scouts check several sites, often abandoning their own original site to promote the superior site of another scout. Several different sites may be promoted by different scouts at first. After some hours and sometimes days, a preferred location eventually emerges from this decision-making process. When all scouts agree on the final location, the whole cluster takes off and swarms to it. Sometimes, if no decision is reached, the swarm will separate, some bees going in one direction; others, going in another. This usually results in failure, with both groups dying. A new location is typically a kilometre or more from the original hive, though some species, e.g., Apis dorsata,[72] may establish new colonies within as little as 500 meters from the natal nest. This collective decision-making process is remarkably successful in identifying the most suitable new nest site and keeping the swarm intact. A good hive site has to be large enough to accommodate the swarm (about 15 litres in volume), has to be well-protected from the elements, receive an optimal amount of sunshine, be some height above the ground, have a small entrance and be capable of resisting ant infestation - that is why tree cavities are often selected.[73][74][75][76][77]
Non-social insects
Unlike social insects, swarms of non-social insects that have been studied primarily seem to function in contexts such as mating, feeding, predator avoidance, and migration.
Moths
Moths may exhibit synchronized mating, during which pheromones released by females initiate searching and swarming behavior in males.[78] Males sense pheromones with sensitive antennae and may track females as far as several kilometers away.[79] Swarm mating involves female choice and male competition. Only one male in the swarm—typically the first—will successfully copulate.[80] Females maximize fitness benefits and minimize cost by governing the onset and magnitude of pheromone deployed. Too little pheromone will not attract a mate, too much allows less fit males to sense the signal.[81] After copulation, females lay the eggs on a host plant. Quality of host plant may be a factor influencing the location of swarming and egg-laying. In one case, researchers observed pink-striped oakworm moths (Anisota virginiensis) swarming at a carrion site, where decomposition likely increased soil nutrient levels and host plant quality.[82]
Flies
Midges, such as Tokunagayusurika akamusi, form swarms, dancing in the air. Swarming serves multiple purposes, including the facilitation of mating by attracting females to approach the swarm, a phenomenon known as lek mating. Such cloud-like swarms often form in early evening when the sun is getting low, at the tip of a bush, on a hilltop, over a pool of water, or even sometimes above a person. The forming of such swarms is not out of instinct, but an adaptive behavior – a "consensus" – between the individuals within the swarms. It is also suggested that swarming is a ritual, because there is rarely any male midge by itself and not in a swarm. This could have formed due to the benefit of lowering inbreeding by having males of various genes gathering in one spot.[83] The genus Culicoides, also known as biting midges, have displayed swarming behavior which are believed to cause confusion in predators.[84]
Cockroaches
Cockroaches leave chemical trails in their feces as well as emitting airborne pheromones for mating. Other cockroaches will follow these trails to discover sources of food and water, and also discover where other cockroaches are hiding. Thus, groups of cockroaches can exhibit emergent behaviour,[85] in which group or swarm behaviour emerges from a simple set of individual interactions.
Cockroaches are mainly nocturnal and will run away when exposed to light. A study tested the hypothesis that cockroaches use just two pieces of information to decide where to go under those conditions: how dark it is and how many other cockroaches there are. The study conducted by José Halloy and colleagues at the Free University of Brussels and other European institutions created a set of tiny robots that appear to the roaches as other roaches and can thus alter the roaches' perception of critical mass. The robots were also specially scented so that they would be accepted by the real roaches.[86]
Locusts

Locusts are the swarming phase of the short-horned grasshoppers of the family Acrididae. Some species can breed rapidly under suitable conditions and subsequently become gregarious and migratory. They form bands as nymphs and swarms as adults—both of which can travel great distances, rapidly stripping fields and greatly damaging crops. The largest swarms can cover hundreds of square miles and contain billions of locusts. A locust can eat its own weight (about 2 grams) in plants every day. That means one million locusts can eat more than one tonne of food each day, and the largest swarms can consume over 100,000 tonnes each day.[87]
Swarming in locusts has been found to be associated with increased levels of serotonin which causes the locust to change colour, eat much more, become mutually attracted, and breed much more easily. Researchers propose that swarming behaviour is a response to overcrowding and studies have shown that increased tactile stimulation of the hind legs or, in some species, simply encountering other individuals causes an increase in levels of serotonin. The transformation of the locust to the swarming variety can be induced by several contacts per minute over a four-hour period.[88][89][90][91] Notably, an innate predisposition to aggregate has been found in hatchlings of the desert locust, Schistocerca gregaria, independent of their parental phase.[92]
An individual locust's response to a loss of alignment in the group appears to increase the randomness of its motion, until an aligned state is again achieved. This noise-induced alignment appears to be an intrinsic characteristic of collective coherent motion.[93]
Migratory behavior

Insect migration is the seasonal movement of insects, particularly those by species of dragonflies, beetles, butterflies, and moths. The distance can vary from species to species, but in most cases these movements involve large numbers of individuals. In some cases the individuals that migrate in one direction may not return and the next generation may instead migrate in the opposite direction. This is a significant difference from bird migration.
Monarch butterflies are especially noted for their lengthy annual migration. In North America they make massive southward migrations starting in August until the first frost. A northward migration takes place in the spring. The monarch is the only butterfly that migrates both north and south as the birds do on a regular basis. But no single individual makes the entire round trip. Female monarchs deposit eggs for the next generation during these migrations.[94] The length of these journeys exceeds the normal lifespan of most monarchs, which is less than two months for butterflies born in early summer. The last generation of the summer enters into a non-reproductive phase known as diapause and may live seven months or more.[95] During diapause, butterflies fly to one of many overwintering sites. The generation that overwinters generally does not reproduce until it leaves the overwintering site sometime in February and March. It is the second, third and fourth generations that return to their northern locations in the United States and Canada in the spring. How the species manages to return to the same overwintering spots over a gap of several generations is still a subject of research; the flight patterns appear to be inherited, based on a combination of the position of the sun in the sky[96] and a time-compensated Sun compass that depends upon a circadian clock that is based in their antennae.[97][98]
Birds


Bird migration
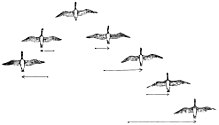
Approximately 1800 of the world's 10,000 bird species are long-distance migrants.[100] The primary motivation for migration appears to be food; for example, some hummingbirds choose not to migrate if fed through the winter. Also, the longer days of the northern summer provide extended time for breeding birds to feed their young. This helps diurnal birds to produce larger clutches than related non-migratory species that remain in the tropics. As the days shorten in autumn, the birds return to warmer regions where the available food supply varies little with the season. These advantages offset the high stress, physical exertion costs, and other risks of the migration such as predation.
Many birds migrate in flocks. For larger birds, it is assumed that flying in flocks reduces energy costs. The V formation is often supposed to boost the efficiency and range of flying birds, particularly over long migratory routes. All the birds except the first fly in the upwash from one of the wingtip vortices of the bird ahead. The upwash assists each bird in supporting its own weight in flight, in the same way a glider can climb or maintain height indefinitely in rising air. Geese flying in a V formation save energy by flying in the updraft of the wingtip vortex generated by the previous animal in the formation. Thus, the birds flying behind do not need to work as hard to achieve lift. Studies show that birds in a V formation place themselves roughly at the optimum distance predicted by simple aerodynamic theory.[101] Geese in a V-formation may conserve 12–20% of the energy they would need to fly alone.[102][103] Red knots and dunlins were found in radar studies to fly 5 km per hour faster in flocks than when they were flying alone.[104] The birds flying at the tips and at the front are rotated in a timely cyclical fashion to spread flight fatigue equally among the flock members. The formation also makes communication easier and allows the birds to maintain visual contact with each other.
External videos | |
---|---|
![]() – from The Trials of Life |
Other animals may use similar drafting techniques when migrating. Lobsters, for example, migrate in close single-file formation "lobster trains", sometimes for hundreds of miles.
The Mediterranean and other seas present a major obstacle to soaring birds, which must cross at the narrowest points. Massive numbers of large raptors and storks pass through areas such as Gibraltar, Falsterbo, and the Bosphorus at migration times. More common species, such as the European honey buzzard, can be counted in hundreds of thousands in autumn. Other barriers, such as mountain ranges, can also cause funnelling, particularly of large diurnal migrants. This is a notable factor in the Central American migratory bottleneck. This concentration of birds during migration can put species at risk. Some spectacular migrants have already gone extinct, the most notable being the passenger pigeon. During migration the flocks were a mile (1.6 km) wide and 300 miles (500 km) long, taking several days to pass and containing up to a billion birds.
Marine life
Fish

External image | |
---|---|
![]() |
The term "shoal" can be used to describe any group of fish, including mixed-species groups, while "school" is used for more closely knit groups of the same species swimming in a highly synchronised and polarised manner.
Fish derive many benefits from shoaling behaviour including defence against predators (through better predator detection and by diluting the chance of capture), enhanced foraging success, and higher success in finding a mate.[106] It is also likely that fish benefit from shoal membership through increased hydrodynamic efficiency.[107]
Fish use many traits to choose shoalmates. Generally they prefer larger shoals, shoalmates of their own species, shoalmates similar in size and appearance to themselves, healthy fish, and kin (when recognised). The "oddity effect" posits that any shoal member that stands out in appearance will be preferentially targeted by predators. This may explain why fish prefer to shoal with individuals that resemble them. The oddity effect would thus tend to homogenise shoals.[108]
One puzzling aspect of shoal selection is how a fish can choose to join a shoal of animals similar to themselves, given that it cannot know its own appearance. Experiments with zebrafish have shown that shoal preference is a learned ability, not innate. A zebrafish tends to associate with shoals that resemble shoals in which it was reared, a form of imprinting.[109]
Other open questions of shoaling behaviour include identifying which individuals are responsible for the direction of shoal movement. In the case of migratory movement, most members of a shoal seem to know where they are going. In the case of foraging behaviour, captive shoals of golden shiner (a kind of minnow) are led by a small number of experienced individuals who knew when and where food was available.[110]
Radakov estimated herring schools in the North Atlantic can occupy up to 4.8 cubic kilometres (1.2 cu mi) with fish densities between 0.5 and 1.0 fish/cubic metre, totalling several billion fish in one school.[111]
- Partridge BL (1982) "The structure and function of fish schools" Scientific American, June:114–123.
- Parrish JK, Viscido SV, Grunbaum D (2002). "Self-Organized Fish Schools: An Examination of Emergent Properties" (PDF). Biol. Bull. 202 (3): 296–305. CiteSeerX 10.1.1.116.1548. doi:10.2307/1543482. JSTOR 1543482. PMID 12087003. S2CID 377484. Archived from the original (PDF) on 11 September 2006.
Fish migration
External image | |
---|---|
![]() |
Between May and July huge numbers of sardines spawn in the cool waters of the Agulhas Bank and then follow a current of cold water northward along the east coast of South Africa. This great migration, called the sardine run, creates spectacular feeding frenzies along the coastline as marine predators, such as dolphins, sharks and gannets attack the schools.
Krill
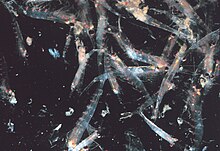
Most krill, small shrimp-like crustaceans, form large swarms, sometimes reaching densities of 10,000–60,000 individual animals per cubic metre.[113][114][115] Swarming is a defensive mechanism, confusing smaller predators that would like to pick out single individuals. The largest swarms are visible from space and can be tracked by satellite.[116] One swarm was observed to cover an area of 450 square kilometres (175 square miles) of ocean, to a depth of 200 meters (650 feet) and was estimated to contain over 2 million tons of krill.[117] Recent research suggests that krill do not simply drift passively in these currents but actually modify them.[117] Krill typically follow a diurnal vertical migration. By moving vertically through the ocean on a 12-hour cycle, the swarms play a major part in mixing deeper, nutrient-rich water with nutrient-poor water at the surface.[117] Until recently it has been assumed that they spend the day at greater depths and rise during the night toward the surface. It has been found that the deeper they go, the more they reduce their activity,[118] apparently to reduce encounters with predators and to conserve energy.
Later work suggested that swimming activity in krill varied with stomach fullness. Satiated animals that had been feeding at the surface swim less actively and therefore sink below the mixed layer.[119] As they sink they produce faeces which may mean that they have an important role to play in the Antarctic carbon cycle. Krill with empty stomachs were found to swim more actively and thus head towards the surface. This implies that vertical migration may be a bi- or tri-daily occurrence. Some species form surface swarms during the day for feeding and reproductive purposes even though such behaviour is dangerous because it makes them extremely vulnerable to predators.[120] Dense swarms may elicit a feeding frenzy among fish, birds and mammal predators, especially near the surface. When disturbed, a swarm scatters, and some individuals have even been observed to moult instantaneously, leaving the exuvia behind as a decoy.[121] In 2012, Gandomi and Alavi presented what appears to be a successful stochastic algorithm for modelling the behaviour of krill swarms. The algorithm is based on three main factors: " (i) movement induced by the presence of other individuals (ii) foraging activity, and (iii) random diffusion."[122]
Copepods

Copepods are a group of tiny crustaceans found in the sea and lakes. Many species are planktonic (drifting in sea waters), and others are benthic (living on the ocean floor). Copepods are typically 1 to 2 millimetres (0.04 to 0.08 in) long, with a teardrop shaped body and large antennae. Although like other crustaceans they have an armoured exoskeleton, they are so small that in most species this thin armour, and the entire body, is almost totally transparent. Copepods have a compound, median single eye, usually bright red, in the centre of the transparent head.
Copepods also swarm. For example, monospecific swarms have been observed regularly around coral reefs and sea grass, and in lakes. Swarms densities were about one million copepods per cubic metre. Typical swarms were one or two metres in diameter, but some exceeded 30 cubic metres. Copepods need visual contact to keep together, and they disperse at night.[123]
Spring produces blooms of swarming phytoplankton which provide food for copepods. Planktonic copepods are usually the dominant members of the zooplankton, and are in turn major food organisms for many other marine animals. In particular, copepods are prey to forage fish and jellyfish, both of which can assemble in vast, million-strong swarms. Some copepods have extremely fast escape responses when a predator is sensed and can jump with high speed over a few millimetres (see animated image below).
- Photo: School of herrings ram feeding on a swarm of copepods.
- Animation showing how herrings hunting in a synchronised way can capture the very alert and evasive copepod (click to view).
- Swarms of jellyfish also prey on copepods
Planktonic copepods are important to the carbon cycle. Some scientists say they form the largest animal biomass on earth.[124] They compete for this title with Antarctic krill. Because of their smaller size and relatively faster growth rates, however, and because they are more evenly distributed throughout more of the world's oceans, copepods almost certainly contribute far more to the secondary productivity of the world's oceans, and to the global ocean carbon sink than krill, and perhaps more than all other groups of organisms together. The surface layers of the oceans are currently believed to be the world's largest carbon sink, absorbing about 2 billion tonnes of carbon a year, the equivalent to perhaps a third of human carbon emissions, thus reducing their impact. Many planktonic copepods feed near the surface at night, then sink into deeper water during the day to avoid visual predators. Their moulted exoskeletons, faecal pellets and respiration at depth all bring carbon to the deep sea.
Algal blooms
Many single-celled organisms called phytoplankton live in oceans and lakes. When certain conditions are present, such as high nutrient or light levels, these organisms reproduce explosively. The resulting dense swarm of phytoplankton is called an algal bloom. Blooms can cover hundreds of square kilometres and are easily seen in satellite images. Individual phytoplankton rarely live more than a few days, but blooms can last weeks.[125][126]
Plants
Scientists have attributed swarm behavior to plants for hundreds of years. In his 1800 book, Phytologia: or, The philosophy of agriculture and gardening, Erasmus Darwin wrote that plant growth resembled swarms observed elsewhere in nature.[127] While he was referring to more broad observations of plant morphology, and was focused on both root and shoot behavior, recent research has supported this claim.
Plant roots, in particular, display observable swarm behavior, growing in patterns that exceed the statistical threshold for random probability, and indicate the presence of communication between individual root apexes. The primary function of plant roots is the uptake of soil nutrients, and it is this purpose which drives swarm behavior. Plants growing in close proximity have adapted their growth to assure optimal nutrient availability. This is accomplished by growing in a direction that optimizes the distance between nearby roots, thereby increasing their chance of exploiting untapped nutrient reserves. The action of this behavior takes two forms: maximization of distance from, and repulsion by, neighboring root apexes.[128] The transition zone of a root tip is largely responsible for monitoring for the presence of soil-borne hormones, signaling responsive growth patterns as appropriate. Plant responses are often complex, integrating multiple inputs to inform an autonomous response. Additional inputs that inform swarm growth includes light and gravity, both of which are also monitored in the transition zone of a root's apex.[129] These forces act to inform any number of growing "main" roots, which exhibit their own independent releases of inhibitory chemicals to establish appropriate spacing, thereby contributing to a swarm behavior pattern. Horizontal growth of roots, whether in response to high mineral content in soil or due to stolon growth, produces branched growth that establish to also form their own, independent root swarms.[130]
Bacteria
Swarming also describes groupings of some kinds of predatory bacteria such as myxobacteria. Myxobacteria swarm together in "wolf packs", actively moving using a process known as bacterial gliding and keeping together with the help of intercellular molecular signals.[57][131]
Mammals


- Parrish JK, Edelstein-Keshet L (1999). "Complexity, pattern and evolutionary trade-offs in animal aggregation" (PDF). Science. 284 (5411): 99–101. Bibcode:1999Sci...284...99P. CiteSeerX 10.1.1.560.5229. doi:10.1126/science.284.5411.99. PMID 10102827. Archived from the original (PDF) on 20 July 2011.
People

External images | |
---|---|
![]() | |
![]() |
A collection of people can also exhibit swarm behaviour, such as pedestrians[134] or soldiers swarming the parapets[dubious – discuss]. In Cologne, Germany, two biologists from the University of Leeds demonstrated flock like behaviour in humans. The group of people exhibited similar behavioural pattern to a flock, where if five percent of the flock changed direction the others would follow. If one person was designated as a predator and everyone else was to avoid him, the flock behaved very much like a school of fish.[135][136] Understanding how humans interact in crowds is important if crowd management is to effectively avoid casualties at football grounds, music concerts and subway stations.[137]
The mathematical modelling of flocking behaviour is a common technology, and has found uses in animation. Flocking simulations have been used in many films[138] to generate crowds which move realistically. Tim Burton's Batman Returns was the first movie to make use of swarm technology for rendering, realistically depicting the movements of a group of bats using the boids system. The Lord of the Rings film trilogy made use of similar technology, known as Massive, during battle scenes. Swarm technology is particularly attractive because it is cheap, robust, and simple.
An ant-based computer simulation using only six interaction rules has also been used to evaluate aircraft boarding behaviour.[139] Airlines have also used ant-based routing in assigning aircraft arrivals to airport gates. An airline system developed by Douglas A. Lawson uses swarm theory, or swarm intelligence—the idea that a colony of ants works better than one alone. Each pilot acts like an ant searching for the best airport gate. "The pilot learns from his experience what's the best for him, and it turns out that that's the best solution for the airline," Lawson explains. As a result, the "colony" of pilots always go to gates they can arrive and depart quickly. The program can even alert a pilot of plane back-ups before they happen. "We can anticipate that it's going to happen, so we'll have a gate available," says Lawson.[140]
Swarm behaviour occurs also in traffic flow dynamics, such as the traffic wave. Bidirectional traffic can be observed in ant trails.[141][142] In recent years this behaviour has been researched for insight into pedestrian and traffic models.[143][144] Simulations based on pedestrian models have also been applied to crowds which stampede because of panic.[145]
Herd behaviour in marketing has been used to explain the dependencies of customers' mutual behaviour. The Economist reported a recent conference in Rome on the subject of the simulation of adaptive human behaviour.[146] It shared mechanisms to increase impulse buying and get people "to buy more by playing on the herd instinct." The basic idea is that people will buy more of products that are seen to be popular, and several feedback mechanisms to get product popularity information to consumers are mentioned, including smart card technology and the use of Radio Frequency Identification Tag technology. A "swarm-moves" model was introduced by a Florida Institute of Technology researcher, which is appealing to supermarkets because it can "increase sales without the need to give people discounts."
- Helbing D, Keltsch J, Molnar P (1997). "Modelling the evolution of human trail systems". Nature. 388 (6637): 47–50. arXiv:cond-mat/9805158. Bibcode:1997Natur.388...47H. doi:10.1038/40353. PMID 9214501. S2CID 4364517.
- Helbing D, Farkas I, Vicsek T (2000). "Simulating dynamical features of escape panic". Nature. 407 (6803): 487–490. arXiv:cond-mat/0009448. Bibcode:2000Natur.407..487H. doi:10.1038/35035023. PMID 11028994. S2CID 310346.
- Helbing D, Farkas IJ, Vicsek T (2000). "Freezing by heating in a driven mesoscopic system". Physical Review Letters. 84 (6): 1240–1243. arXiv:cond-mat/9904326. Bibcode:2000PhRvL..84.1240H. doi:10.1103/PhysRevLett.84.1240. PMID 11017488. S2CID 18649078.
Robotics
The application of swarm principles to robots is called swarm robotics, while swarm intelligence refers to the more general set of algorithms.
External videos | |
---|---|
![]() | |
![]() |
Partially inspired by colonies of insects such as ants and bees, researchers are modelling the behaviour of swarms of thousands of tiny robots which together perform a useful task, such as finding something hidden, cleaning, or spying. Each robot is quite simple, but the emergent behaviour of the swarm is more complex.[1] The whole set of robots can be considered as one single distributed system, in the same way an ant colony can be considered a superorganism, exhibiting swarm intelligence. The largest swarms so far created is the 1024 robot Kilobot swarm.[148] Other large swarms include the iRobot swarm, the SRI International/ActivMedia Robotics Centibots project,[149] and the Open-source Micro-robotic Project swarm, which are being used to research collective behaviours.[150][151] Swarms are also more resistant to failure. Whereas one large robot may fail and ruin a mission, a swarm can continue even if several robots fail. This could make them attractive for space exploration missions, where failure is normally extremely costly.[152] In addition to ground vehicles, swarm robotics includes also research of swarms of aerial robots[147][153] and heterogeneous teams of ground and aerial vehicles.[154][155]
In contrast macroscopic robots, colloidal particles at microscale can also be adopted as agents to perform collective behaviors to conduct tasks using mechanical and physical approaches, such as reconfigurable tornado-like microswarm[156] mimicking schooling fish,[157] hierarchical particle species[158] mimicking predating behavior of mammals, micro-object manipulation using a transformable microswarm.[159] The fabrication of such colloidal particles is usually based on chemical synthesis.
Military

Military swarming is a behaviour where autonomous or partially autonomous units of action attack an enemy from several different directions and then regroup. Pulsing, where the units shift the point of attack, is also a part of military swarming. Military swarming involves the use of a decentralized force against an opponent, in a manner that emphasizes mobility, communication, unit autonomy and coordination or synchronization.[160] Historically military forces used principles of swarming without really examining them explicitly, but now active research consciously examines military doctrines that draw ideas from swarming.
Merely because multiple units converge on a target, they are not necessarily swarming. Siege operations do not involve swarming, because there is no manoeuvre; there is convergence but on the besieged fortification. Nor do guerrilla ambushes constitute swarms, because they are "hit-and-run". Even though the ambush may have several points of attack on the enemy, the guerillas withdraw when they either have inflicted adequate damage, or when they are endangered.
In 2014 the U. S. Office of Naval Research released a video showing tests of a swarm of small autonomous drone attack boats that can steer and take coordinated offensive action as a group.[161]
Gallery
- A swarm of migrating herrings
- A swarm of bees
- A swarm of ladybirds
- A swarm of robots
- A swarm of ancient stars
Myths
- There is a popular myth that lemmings commit mass suicide by swarming off cliffs when they migrate. Driven by strong biological urges, some species of lemmings may migrate in large groups when population density becomes too great. Lemmings can swim and may choose to cross a body of water in search of a new habitat. In such cases, many may drown if the body of water is so wide as to stretch their physical capability to the limit. This fact combined with some unexplained fluctuations in the population of Norwegian lemmings gave rise to the myth.[165]
- Piranha have a reputation as fearless fish that swarm in ferocious and predatory packs. However, recent research, which started "with the premise that they school as a means of cooperative hunting", discovered that they were in fact rather fearful fish, like other fish, who schooled for protection from their predators, such as cormorants, caimans and dolphins. A researcher described them as "basically like regular fish with large teeth".[166]
See also
- Active matter – Matter behavior at system scale
- Dyson swarm – Hypothetical megastructure around a star
- List of collective nouns in English
- Mobile Bay jubilee – Natural phenomenon that occurs in Mobile Bay, Alabama, United States
- Population cycle – the repeated increase and decrease of a species' population over time
- Swarm (simulation) – open-source agent-based simulation toolkit
- Swirlonic state – recently (2020) recognised new state of self propelled particles
- Traffic wave – Type of highway congestion
- Swarmalators - Agents that do swarming and synchronization simultaneously.
References
- ^ a b Bouffanais, Roland (2016). Design and Control of Swarm Dynamics. SpringerBriefs in Complexity (First ed.). Springer. doi:10.1007/978-981-287-751-2. ISBN 978-981-287-750-5.
- ^ O'Loan; Evans (1998). "Alternating steady state in one-dimensional flocking". Journal of Physics A: Mathematical and General. 32 (8): L99 – L105. arXiv:cond-mat/9811336. Bibcode:1999JPhA...32L..99O. doi:10.1088/0305-4470/32/8/002. S2CID 7642063.
- ^ Attanasi, A.; Cavagna, A.; Del Castello, L.; Giardina, I.; Grigera, T. S.; Jelić, A.; Melillo, S.; Parisi, L.; Pohl, O.; Shen, E.; Viale, M. (September 2014). "Information transfer and behavioural inertia in starling flocks". Nature Physics. 10 (9): 691–696. arXiv:1303.7097. Bibcode:2014NatPh..10..691A. doi:10.1038/nphys3035. PMC 4173114. PMID 25264452.
- ^ a b c Reynolds CW (1987). "Flocks, herds and schools: A distributed behavioral model". Proceedings of the 14th annual conference on Computer graphics and interactive techniques. Vol. 21. pp. 25–34. CiteSeerX 10.1.1.103.7187. doi:10.1145/37401.37406. ISBN 978-0-89791-227-3. S2CID 546350.
- ^ a b Ballerini M, Cabibbo N, Candelier R, Cavagna A, Cisbani E, Giardina I, Lecomte V, Orlandi A, Parisi G, Procaccini A, Viale M, Zdravkovic V (2008). "Interaction ruling animal collective behavior depends on topological rather than metric distance: Evidence from a field study". Proc. Natl. Acad. Sci. U.S.A. 105 (4): 1232–7. arXiv:0709.1916. Bibcode:2008PNAS..105.1232B. doi:10.1073/pnas.0711437105. PMC 2234121. PMID 18227508.
- ^ Hildenbrandt H, Carere C, Hemelrijk CK (2010). "Self-organized aerial displays of thousands of starlings: a model". Behavioral Ecology. 21 (6): 1349–1359. arXiv:0908.2677. doi:10.1093/beheco/arq149.
- ^ Hemelrijk CK, Hildenbrandt H (2011). "Some causes of the variable shape of flocks of birds". PLOS ONE. 6 (8): e22479. Bibcode:2011PLoSO...622479H. doi:10.1371/journal.pone.0022479. PMC 3150374. PMID 21829627.
- ^ "Zwermen en scholen - Swarming - Permanente expo - Bezoek onze expo's & workshops! - Science LinX - Rijksuniversiteit Groningen". 10 November 2007.
- ^ "Onderzoek aan de Faculteit Wiskunde en Natuurwetenschappen - Faculteit Wiskunde en Natuurwetenschappen - Over ons - Rijksuniversiteit Groningen". 25 October 2012.
- ^ Yang, W.; Schmickl, T. (2019). "Collective Motion as an Ultimate Effect in Crowded Selfish Herds". Scientific Reports. 9 (1): 6618. Bibcode:2019NatSR...9.6618Y. doi:10.1038/s41598-019-43179-6. PMC 6488663. PMID 31036873.
- ^ Olson RS, Knoester DB, Adami C (2013). "Critical interplay between density-dependent predation and evolution of the selfish herd". Proceedings of the 15th annual conference on Genetic and evolutionary computation. Gecco '13. pp. 247–254. doi:10.1145/2463372.2463394. ISBN 9781450319638. S2CID 14414033.
- ^ Ward CR, Gobet F, Kendall G (2001). "Evolving collective behavior in an artificial ecology". Artificial Life. 7 (2): 191–209. CiteSeerX 10.1.1.108.3956. doi:10.1162/106454601753139005. PMID 11580880. S2CID 12133884.
- ^ Reluga TC, Viscido S (2005). "Simulated evolution of selfish herd behavior". Journal of Theoretical Biology. 234 (2): 213–225. Bibcode:2005JThBi.234..213R. doi:10.1016/j.jtbi.2004.11.035. PMID 15757680.
- ^ Wood AJ, Ackland GJ (2007). "Evolving the selfish herd: emergence of distinct aggregating strategies in an individual-based model". Proc Biol Sci. 274 (1618): 1637–1642. doi:10.1098/rspb.2007.0306. PMC 2169279. PMID 17472913.
- ^ Olson RS, Hintze A, Dyer FC, Knoester DB, Adami C (2013). "Predator confusion is sufficient to evolve swarming behaviour". J. R. Soc. Interface. 10 (85): 20130305. doi:10.1098/rsif.2013.0305. PMC 4043163. PMID 23740485.
- ^ Demsar J, Hemelrijk CK, Hildenbrandt H, Bajec IL (2015). "Simulating predator attacks on schools: Evolving composite tactics" (PDF). Ecological Modelling. 304: 22–33. Bibcode:2015EcMod.304...22D. doi:10.1016/j.ecolmodel.2015.02.018. hdl:11370/0bfcbb69-a101-4ec1-833a-df301e49d8ef. S2CID 46988508.
- ^ Tosh CR (2011). "Which conditions promote negative density dependent selection on prey aggregations?" (PDF). Journal of Theoretical Biology. 281 (1): 24–30. Bibcode:2011JThBi.281...24T. doi:10.1016/j.jtbi.2011.04.014. PMID 21540037.
- ^ Ioannou CC, Guttal V, Couzin ID (2012). "Predatory Fish Select for Coordinated Collective Motion in Virtual Prey". Science. 337 (6099): 1212–1215. Bibcode:2012Sci...337.1212I. doi:10.1126/science.1218919. PMID 22903520. S2CID 10203872.
- ^ Olson RS, Haley PB, Dyer FC, Adami C (2015). "Exploring the evolution of a trade-off between vigilance and foraging in group-living organisms". Royal Society Open Science. 2 (9): 150135. arXiv:1408.1906. Bibcode:2015RSOS....250135O. doi:10.1098/rsos.150135. PMC 4593673. PMID 26473039.
- ^ "Hierarchy of Life". 14 September 2008. Archived from the original on 3 July 2016. Retrieved 6 October 2009.
- ^ Parunak, H. v D. (2003). "Making swarming happen" In: Proceedings of Conference on Swarming and Network Enabled Command, Control, Communications, Computers, Intelligence, Surveillance and Reconnaissance (C4ISR), McLean, Virginia, USA, 3 January 2003.
- ^ a b Marsh L.; Onof C. (2008). "Stigmergic epistemology, stigmergic cognition" (PDF). Cognitive Systems Research. 9 (1): 136–149. doi:10.1016/j.cogsys.2007.06.009. S2CID 23140721.
- ^ Beni, G., Wang, J. Swarm Intelligence in Cellular Robotic Systems, Proceed. NATO Advanced Workshop on Robots and Biological Systems, Tuscany, Italy, June 26–30 (1989)
- ^ Dorigo, M; Birattari, M (2007). "Swarm intelligence". Scholarpedia. 2 (9): 1462. Bibcode:2007SchpJ...2.1462D. doi:10.4249/scholarpedia.1462.
- ^ a b Li, YX; Lukeman, R; Edelstein-Keshet, L (2007). "Minimal mechanisms for school formation in self-propelled particles" (PDF). Physica D: Nonlinear Phenomena. 237 (5): 699–720. Bibcode:2008PhyD..237..699L. doi:10.1016/j.physd.2007.10.009.
- ^ Toner J and Tu Y (1995) "Long-range order in a two-dimensional xy model: how birds fly together" Physical Revue Letters, 75 (23)(1995), 4326–4329.
- ^ Topaz C, Bertozzi A (2004). "Swarming patterns in a two-dimensional kinematic model for biological groups". SIAM J Appl Math. 65 (1): 152–174. Bibcode:2004APS..MAR.t9004T. CiteSeerX 10.1.1.88.3071. doi:10.1137/S0036139903437424. S2CID 18468679.
- ^ Topaz C, Bertozzi A, Lewis M (2006). "A nonlocal continuum model for biological aggregation". Bull Math Biol. 68 (7): 1601–1623. arXiv:q-bio/0504001. doi:10.1007/s11538-006-9088-6. PMID 16858662. S2CID 14750061.
- ^ Carrillo, J; Fornasier, M; Toscani, G (2010). "Particle, kinetic, and hydrodynamic models of swarming" (PDF). Mathematical Modeling of Collective Behavior in Socio-Economic and Life Sciences. Modeling and Simulation in Science, Engineering and Technology. Vol. 3. pp. 297–336. CiteSeerX 10.1.1.193.5047. doi:10.1007/978-0-8176-4946-3_12. ISBN 978-0-8176-4945-6.
- ^ "Swarmanoid project". Archived from the original on 5 July 2007.
- ^ Ant colony optimization Retrieved 15 December 2010.
- ^ A. Colorni, M. Dorigo et V. Maniezzo, Distributed Optimization by Ant Colonies, actes de la première conférence européenne sur la vie artificielle, Paris, Elsevier Publishing, 134–142, 1991.
- ^ M. Dorigo, Optimization, Learning and Natural Algorithms, PhD thesis, Politecnico di Milano, Italie, 1992.
- ^ Hölldobler & Wilson (1990), pp. 143–179
- ^ DORIGO, M.; DI CARO, G.; GAMBERELLA, L. M. (1999). Ant Algorithms for Discrete Optimization, Artificial Life. MIT Press.
- ^ Self driven particle model Archived 2012-10-14 at the Wayback Machine Interactive simulations, 2005, University of Colorado. Retrieved 10 April 2011.
- ^ a b Vicsek T, Czirok A, Ben-Jacob E, Cohen I, Shochet O (1995). "Novel type of phase transition in a system of self-driven particles". Physical Review Letters. 75 (6): 1226–1229. arXiv:cond-mat/0611743. Bibcode:1995PhRvL..75.1226V. doi:10.1103/PhysRevLett.75.1226. PMID 10060237. S2CID 15918052.
- ^ Czirók A, Vicsek T (2006). "Collective behavior of interacting self-propelled particles". Physica A. 281 (1–4): 17–29. arXiv:cond-mat/0611742. Bibcode:2000PhyA..281...17C. doi:10.1016/S0378-4371(00)00013-3. S2CID 14211016.
- ^ Buhl J, Sumpter DJT, Couzin D, Hale JJ, Despland E, Miller ER, Simpson SJ, et al. (2006). "From disorder to order in marching locusts" (PDF). Science. 312 (5778): 1402–1406. Bibcode:2006Sci...312.1402B. doi:10.1126/science.1125142. PMID 16741126. S2CID 359329. Archived from the original (PDF) on 29 September 2011. Retrieved 13 April 2011.
- ^ Toner J, Tu Y, Ramaswamy S (2005). "Hydrodynamics and phases of flocks" (PDF). Annals of Physics. 318 (1): 170–244. Bibcode:2005AnPhy.318..170T. doi:10.1016/j.aop.2005.04.011. Archived from the original (PDF) on 18 July 2011. Retrieved 13 April 2011.
- ^ Bertin, E; Droz; Grégoire, G (2009). "Hydrodynamic equations for self-propelled particles: microscopic derivation and stability analysis". J. Phys. A. 42 (44): 445001. arXiv:0907.4688. Bibcode:2009JPhA...42R5001B. doi:10.1088/1751-8113/42/44/445001. S2CID 17686543.
- ^ Kennedy, J.; Eberhart, R. (1995). "Particle Swarm Optimization". Proceedings of IEEE International Conference on Neural Networks. Vol. IV. pp. 1942–1948.
- ^ Kennedy, J. (1997). "The particle swarm: social adaptation of knowledge". Proceedings of IEEE International Conference on Evolutionary Computation. pp. 303–308.
- ^ Hu X Particle swarm optimization: Tutorial. Retrieved 15 December 2010.
- ^ Kennedy, J.; Eberhart, R.C. (2001). Swarm Intelligence. Morgan Kaufmann. ISBN 978-1-55860-595-4.
- ^ Poli, R. (2007). "An analysis of publications on particle swarm optimisation applications" (PDF). Technical Report CSM-469. Archived from the original (PDF) on 16 July 2011. Retrieved 15 December 2010.
- ^ Poli, R. (2008). "Analysis of the publications on the applications of particle swarm optimisation" (PDF). Journal of Artificial Evolution and Applications. 2008: 1–10. doi:10.1155/2008/685175.
- ^ Altruism helps swarming robots fly better Archived 2012-09-15 at the Wayback Machine genevalunch.com, 4 May 2011.
- ^ Waibel, M; Floreano, D; Keller, L (2011). "A quantitative test of Hamilton's rule for the evolution of altruism". PLOS Biology. 9 (5): 1000615. doi:10.1371/journal.pbio.1000615. PMC 3086867. PMID 21559320.
- ^ Herrnkind, W (1969). "Queuing behavior of spiny lobsters". Science. 164 (3886): 1425–1427. Bibcode:1969Sci...164.1425H. doi:10.1126/science.164.3886.1425. PMID 5783720. S2CID 10324354.
- ^ Fossil 'conga lines' reveal origins of animal swarms National Geographic, 17 October 2019.
- ^ Vannier, J; Vidal, M; Marchant, R; El Hariri, K; Kouraiss, K; Pittet, B; El Albani, A; Mazurier, A; Martin, E (2019). "Collective behaviour in 480-million-year-old trilobite arthropods from Morocco". Scientific Reports. 9 (1): 14941. Bibcode:2019NatSR...914941V. doi:10.1038/s41598-019-51012-3. PMC 6797724. PMID 31624280.
- ^ Feare C (1984) The Starling, Oxford University Press. ISBN 978-0-19-217705-6.
- ^ Partridge BL (1982). "The structure and function of fish schools" (PDF). Scientific American. Vol. 246, no. 6. pp. 114–123. Bibcode:1982SciAm.246f.114P. doi:10.1038/scientificamerican0682-114. PMID 7201674. Archived from the original (PDF) on 3 July 2011.
- ^ Hubbard S, Babak P, Sigurdsson S, Magnusson K (2004). "A model of the formation of fish schools and migrations of fish". Ecol. Model. 174 (4): 359–374. Bibcode:2004EcMod.174..359H. doi:10.1016/j.ecolmodel.2003.06.006.
- ^ Rauch E, Millonas M, Chialvo D (1995). "Pattern formation and functionality in swarm models". Physics Letters A. 207 (3–4): 185–193. arXiv:adap-org/9507003. Bibcode:1995PhLA..207..185R. doi:10.1016/0375-9601(95)00624-C. S2CID 120567147.
- ^ a b Allison C, Hughes C (1991). "Bacterial swarming: an example of prokaryotic differentiation and multicellular behaviour". Science Progress. 75 (298 Pt 3–4): 403–422. PMID 1842857.
- ^ Ben-Jacob E, Cohen I, Shochet O, Czirok A, Vicsek T (1995). "Cooperative Formation of Chiral Patterns during Growth of Bacterial Colonies". Physical Review Letters. 75 (15): 2899–2902. Bibcode:1995PhRvL..75.2899B. doi:10.1103/PhysRevLett.75.2899. PMID 10059433.
- ^ Rappel WJ, Nicol A, Sarkissian A, Levine H, Loomis WF (1999). "Self-organized vortex state in two-dimensional Dictyostelium dynamics". Physical Review Letters. 83 (6): 1247–1250. arXiv:patt-sol/9811001. Bibcode:1999PhRvL..83.1247R. doi:10.1103/PhysRevLett.83.1247. S2CID 1590827.
- ^ Chowdhury, D (2006). "Collective effects in intra-cellular molecular motor transport: coordination, cooperation and competition". Physica A. 372 (1): 84–95. arXiv:physics/0605053. Bibcode:2006PhyA..372...84C. doi:10.1016/j.physa.2006.05.005. S2CID 14822256.
- ^ Parrish JK and Hamner WM (eds) (1997) Animal Groups in Three Dimensions Cambridge University Press. ISBN 978-0-521-46024-8.
- ^ Helbing D, Keltsch J, Molnar P (1997). "Modelling the evolution of human trail systems". Nature. 388 (6637): 47–50. arXiv:cond-mat/9805158. Bibcode:1997Natur.388...47H. doi:10.1038/40353. PMID 9214501. S2CID 4364517.
- ^ Helbing D, Farkas I, Vicsek T (2000). "Simulating dynamical features of escape panic". Nature. 407 (6803): 487–490. arXiv:cond-mat/0009448. Bibcode:2000Natur.407..487H. doi:10.1038/35035023. PMID 11028994. S2CID 310346.
- ^ Helbing D, Farkas IJ, Vicsek T (2000). "Freezing by heating in a driven mesoscopic system". Physical Review Letters. 84 (6): 1240–1243. arXiv:cond-mat/9904326. Bibcode:2000PhRvL..84.1240H. doi:10.1103/PhysRevLett.84.1240. PMID 11017488. S2CID 18649078.
- ^
- Swarm Theory National Geographic. Feature article, July 2007.
- Beekman M, Sword GA and Simpson SK (2008) Biological Foundations of Swarm Intelligence. In Swarm intelligence: introduction and applications, Eds Blum C and Merkle D. シュプリンガー・ジャパン株式会社, Page 3–43. ISBN 978-3-540-74088-9
- Parrish JK, Edelstein-Keshet L (1999). "Complexity, pattern and evolutionary trade-offs in animal aggregation" (PDF). Science. 284 (5411): 99–101. Bibcode:1999Sci...284...99P. CiteSeerX 10.1.1.560.5229. doi:10.1126/science.284.5411.99. PMID 10102827. Archived from the original (PDF) on 20 July 2011.
- ^ a b Bonabeau E and Theraulaz G (2008) "Swarm Smarts". In Your Future with Robots Scientific American Special Editions.
- ^ Czaczkes, T.J.; Grüter, C.; Ratnieks, F. L. W. (2015). "Trail pheromones: an integrative view of their role in colony organisation". Annual Review of Entomology. 60: 581–599. doi:10.1146/annurev-ento-010814-020627. PMID 25386724. S2CID 37972066.
- ^ Beckers, R.; Deneubourg, J. L.; Goss, S (1993). "Modulation of trail laying in the ant Lasius niger (Hymenoptera: Formicidae) and its role in the collective selection of a food source". Journal of Insect Behavior. 6 (6): 751–759. Bibcode:1993JIBeh...6..751B. CiteSeerX 10.1.1.161.9541. doi:10.1007/BF01201674. S2CID 14670150.
- ^ Goss, S.; Aron, S.; Deneubourg, J. L.; Pasteels, J. M. (1989). "Self-organized shortcuts in the Argentine ant" (PDF). Naturwissenschaften. 76 (12): 579–581. Bibcode:1989NW.....76..579G. doi:10.1007/BF00462870. S2CID 18506807.
- ^ Brady, Seán G. (2003). "Evolution of the army ant syndrome: The origin and long-term evolutionary stasis of a complex of behavioral and reproductive adaptations". Proceedings of the National Academy of Sciences of the United States of America. 100 (11): 6575–9. Bibcode:2003PNAS..100.6575B. doi:10.1073/pnas.1137809100. PMC 164488. PMID 12750466.
- ^ Dicke E, Byde A, Cliff D, Layzell P (2004). "An ant-inspired technique for storage area network design". In A. J. Ispeert, M. Murata, N. Wakamiya (eds.). Proceedings of Biologically Inspired Approaches to Advanced Information Technology: First International Workshop, BioADIT 2004 LNCS 3141. Springer. pp. 364–379. ISBN 9783540233398.
- ^ Oldroyd, BP (1998). "Colony relatedness in aggregations of Apis dorsata Fabricius (Hymenoptera, Apidae)". Insectes Sociaux. 47: 94–95. doi:10.1007/s000400050015. S2CID 40346464.
- ^ Milius, Susan Swarm Savvy Archived 27 September 2012 at the Wayback Machine, How bees, ants and other animals avoid dumb collective decisions; Science News, May 9th, 2009; Vol.175 #10 (p. 16)
- ^ Bee Swarms Follow High-speed 'Streaker' Bees To Find A New Nest; ScienceDaily (Nov. 24, 2008)
- ^ Seeley, Thomas D.; Visscher, P. Kirk (2003). "Choosing a home: how the scouts in a honey bee swarm perceive the completion of their group decision making" (PDF). Behavioral Ecology and Sociobiology. 54 (5): 511–520. Bibcode:2003BEcoS..54..511S. doi:10.1007/s00265-003-0664-6. S2CID 16948152. Archived from the original (PDF) on 31 January 2009. Retrieved 14 December 2010.
- ^ Morse, R.A. (1963). "Swarm orientation in honeybees". Science. 141 (3578): 357–358. Bibcode:1963Sci...141..357M. doi:10.1126/science.141.3578.357. PMID 17815993. S2CID 46516976.
- ^ Seeley, Thomas (2003). "Consensus building during nest-site selection in honey bee swarms: The expiration of dissent". Behavioral Ecology and Sociobiology. 53 (6): 417–424. Bibcode:2003BEcoS..53..417S. doi:10.1007/s00265-003-0598-z. S2CID 34798300.
- ^ Stepien, T.L.; Zmurchok, C.; Hengenius, J.B.; Caja Rivera, R.M.; D'Orsogna, M.R.; Lindsay, A.E. (2000). "Moth Mating: Modeling Female Pheromone Calling and Male Navigational Strategies to Optimize Reproductive Success". Applied Sciences. 10 (18): 6543. doi:10.3390/app10186543.
- ^ Badeke, B.; Haverkamp, A.; Sachse, S.A. (2016). "A challenge for a male noctuid moth? Discerning the female sex pheromone against the background of plant volatiles". Frontiers in Physiology. 7: 143. doi:10.3389/fphys.2016.00143. PMC 4843018. PMID 27199761.
- ^ Greenfield, M.D. (1981). "Moth sex pheromones: an evolutionary perspective". The Florida Entomologist. 64 (1): 4–17. doi:10.2307/3494597. JSTOR 3494597.
- ^ Umbers, K.D.L.; Symonds, M.R.E.; Kokko, H. (2015). "The Mothematics of female pheromone signaling: Strategies for aging virgins" (PDF). American Naturalist. 185 (3): 417–432. doi:10.1086/679614. hdl:1885/13166. PMID 25674695. S2CID 13846468.
- ^ Mason, D.S.; Baruzzi, C. (2019). "Love in strange places". Frontiers in Ecology and the Environment. 17 (3): 184. Bibcode:2019FrEE...17..184M. doi:10.1002/fee.2027.
- ^ "Midges". MDC Discover Nature. Retrieved 19 November 2019.
- ^ Kirkeby, Carsten (30 June 2018). "Observations of female and mixed sex swarming behaviour in Culicoides LATREILLE, 1809 (Diptera: Ceratopogonidae)". Polish Journal of Entomology. 87 (2): 191–197. doi:10.2478/pjen-2018-0014.
- ^ Jennifer Viegas. "Cockroaches Make Group Decisions". Discovery Channel. Archived from the original on 4 July 2008. Retrieved 10 June 2006.
- ^ Lemonick, Michael D. (15 November 2007). "Robotic Roaches Do the Trick". Time. Archived from the original on 16 November 2007.
- ^ Locust Locustidae National Geographic. Retrieved 12 December 2010.
- ^ "Locust swarms 'high' on serotonin". 29 January 2009 – via BBC.
- ^ Rogers SM, Matheson T, Despland E, Dodgson T, Burrows M, Simpson SJ (November 2003). "Mechanosensory-induced behavioural gregarization in the desert locust Schistocerca gregaria". J. Exp. Biol. 206 (Pt 22): 3991–4002. doi:10.1242/jeb.00648. PMID 14555739.
- ^ Stevenson, PA (2009). "The Key to Pandora's Box". Science. 323 (5914): 594–5. doi:10.1126/science.1169280. PMID 19179520. S2CID 39306643.
- ^ Blocking 'happiness' chemical may prevent locust plagues, New Scientist, 2009-01-29, accessed 2009-01-31
- ^ Moshe Guershon; Amir Ayali (May 2012). "Innate phase behavior in the desert locust, Schistocerca gregaria". Insect Science. 19 (6): 649–656. Bibcode:2012InsSc..19..649G. doi:10.1111/j.1744-7917.2012.01518.x. S2CID 83412818.
- ^ Yates, CA; Erban, R; Escudero, C; Couzin, ID; Buhl, J; Kevrekidis, IG; Maini, PK; Sumpter, DJ (2009). "Inherent noise can facilitate coherence in collective swarm motion". Proc. Natl. Acad. Sci. U.S.A. 106 (14): 5464–9. Bibcode:2009PNAS..106.5464Y. doi:10.1073/pnas.0811195106. PMC 2667078. PMID 19336580.
- ^ Pyle, Robert Michael, "National Audubon Society Field Guide to North American Butterflies", p712-713, Alfred A. Knopf, New York, ISBN 0-394-51914-0
- ^ "Monarch, Danaus plexippus". Archived from the original on 15 December 2012. Retrieved 27 August 2008.
- ^ Gugliotta, Guy (2003): Butterflies Guided By Body Clocks, Sun Scientists Shine Light on Monarchs' Pilgrimage Archived 2006-03-05 at the Wayback Machine. Washington Post, May 23, 2003, page A03. Retrieved 2006-JAN-07.
- ^ Merlin C, Gegear RJ, Reppert SM (2009). "Antennal Circadian Clocks Coordinate Sun Compass Orientation in Migratory Monarch Butterflies". Science. 325 (5948): 1700–1704. Bibcode:2009Sci...325.1700M. doi:10.1126/science.1176221. PMC 2754321. PMID 19779201.
- ^ Kyriacou CP (2009). "Unraveling Traveling". Science. 325 (5948): 1629–1630. doi:10.1126/science.1178935. PMID 19779177. S2CID 206522416.
- ^ Nagy, M; Akos Zs, Biro D; Vicsek, T (2010). "Hierarchical group dynamics in pigeon flocks" (PDF). Nature. 464 (7290): 890–893. arXiv:1010.5394. Bibcode:2010Natur.464..890N. doi:10.1038/nature08891. PMID 20376149. S2CID 4430488. Archived from the original (PDF) on 6 July 2010. Supplementary pdf
- ^ Sekercioglu, C.H. (2007). "Conservation ecology: area trumps mobility in fragment bird extinctions". Current Biology. 17 (8): R283 – R286. Bibcode:2007CBio...17.R283S. doi:10.1016/j.cub.2007.02.019. PMID 17437705. S2CID 744140.
- ^ Drag Reduction from Formation Flight. Flying Aircraft in Bird-Like Formations Could Significantly Increase Range; Defense Technical Information Center; April 2002; Retrieved February 27, 2008
- ^ Hummel D.; Beukenberg M. (1989). "Aerodynamische Interferenzeffekte beim Formationsfl ug von Vogeln". J. Ornithol. 130 (1): 15–24. doi:10.1007/BF01647158. S2CID 823269.
- ^ Cutts, C. J. & J R Speakman (1994). "Energy savings in formation flight of Pink-footed Geese" (PDF). J. Exp. Biol. 189 (1): 251–261. doi:10.1242/jeb.189.1.251. PMID 9317742.
- ^ Newton, I. (2008). The Migration Ecology of Birds. Elselvier. ISBN 978-0-12-517367-4.
- ^ Pitcher et al. 1982.
- ^ Pitcher TJ and Parish JK (1993) "Functions of shoaling behaviour in teleosts" In: Pitcher TJ (ed) Behaviour of teleost fishes. Chapman and Hall, New York, pp 363–440
- ^ Hoare DJ, Krause J, Peuhkuri N and Godin JGJ (2000) Body size and shoaling in fish Journal of Fish Biology, 57(6) 1351-1366.
- ^ Snekser JL, Ruhl N, Bauer K, McRobert SP (2010). "The Influence of Sex and Phenotype on Shoaling Decisions in Zebrafish" (PDF). International Journal of Comparative Psychology. 23: 70–81. doi:10.46867/IJCP.2010.23.01.04. Archived from the original (PDF) on 25 July 2011.
- ^ Engeszer RE, Ryan MJ, Parichy DM (2004). "Learned Social Preference in Zebrafish" (PDF). Current Biology. 14 (10): 881–884. Bibcode:2004CBio...14..881E. doi:10.1016/j.cub.2004.04.042. PMID 15186744. S2CID 18741014.
- ^ Reebs, S.G. (2000). "Can a minority of informed leaders determine the foraging movements of a fish shoal?". Animal Behaviour. 59 (2): 403–9. doi:10.1006/anbe.1999.1314. PMID 10675263. S2CID 4945309.
- ^ Radakov DV (1973) Schooling in the ecology of fish. Israel Program for Scientific Translation, translated by Mill H. Halsted Press, New York. ISBN 978-0-7065-1351-6
- ^ Photographer: Mark van Coller
- ^ Hamner, WM; Hamner, PP; Strand, SW; Gilmer, RW (1983). "Behavior of Antarctic Krill, Euphausia superba: Chemoreception, Feeding, Schooling and Molting'". Science. 220 (4595): 433–5. Bibcode:1983Sci...220..433H. doi:10.1126/science.220.4595.433. PMID 17831417. S2CID 22161686.
- ^ U. Kils; P. Marshall (1995). "Der Krill, wie er schwimmt und frisst – neue Einsichten mit neuen Methoden ("The Antarctic krill – how it swims and feeds – new insights with new methods")". In I. Hempel; G. Hempel (eds.). Biologie der Polarmeere – Erlebnisse und Ergebnisse (Biology of the Polar Oceans Experiences and Results). Fischer Verlag. pp. 201–210. ISBN 978-3-334-60950-7.
- ^ R. Piper (2007). Extraordinary Animals: An Encyclopedia of Curious and Unusual Animals. Greenwood Press. ISBN 978-0-313-33922-6.
- ^ Hoare, Ben (2009). Animal Migration. London: Natural History Museum. p. 107. ISBN 978-0-565-09243-6.
- ^ a b c Hoare, Ben (2009). Animal Migration. London: Natural History Museum. p. 107. ISBN 978-0-565-09243-6
- ^ J.S. Jaffe; M.D. Ohmann; A. de Robertis (1999). "Sonar estimates of daytime activity levels of Euphausia pacifica in Saanich Inlet" (PDF). Canadian Journal of Fisheries and Aquatic Sciences. 56 (11): 2000–10. doi:10.1139/cjfas-56-11-2000. S2CID 228567512. Archived from the original (PDF) on 20 July 2011.
- ^ Geraint A. Tarling & Magnus L. Johnson (2006). "Satiation gives krill that sinking feeling". Current Biology. 16 (3): 83–4. Bibcode:2006CBio...16..R83T. doi:10.1016/j.cub.2006.01.044. PMID 16461267.
- ^ Howard, D.: "Krill", pp. 133–140 in Karl, H.A. et al. (eds): Beyond the Golden Gate – Oceanography, Geology, Biology, and Environmental Issues in the Gulf of the Farallones, USGS Circular 1198, 2001. URLs last accessed 2010-06-04.
- ^ D. Howard. "Krill in Cordell Bank National Marine Sanctuary". NOAA. Retrieved 15 June 2005.
- ^ Gandomi, A.H.; Alavi, A.H. (2012). "Krill Herd Algorithm: A New Bio-Inspired Optimization Algorithm". Communications in Nonlinear Science and Numerical Simulation. 17 (12): 4831–4845. Bibcode:2012CNSNS..17.4831G. doi:10.1016/j.cnsns.2012.05.010.
- ^ Hamner, WM; Carleton, JH (1979). "Copepod swarms: Attributes and role in coral reef ecosystems". Limnol. Oceanogr. 24 (1): 1–14. Bibcode:1979LimOc..24....1H. doi:10.4319/lo.1979.24.1.0001.
- ^ Johannes Dürbaum & Thorsten Künnemann (5 November 1997). "Biology of Copepods: An Introduction". Carl von Ossietzky University of Oldenburg. Archived from the original on 26 May 2010. Retrieved 8 December 2009.
- ^ Lindsey R and Scott M (2010) What are phytoplankton NASA Earth Observatory.
- ^ Harmful algal blooms in the Great Lakes Archived 2010-06-16 at the Wayback Machine 2009, NOAA, Center of Excellence for Great Lakes and Human Health.
- ^ Darwin, Erasmus (1 January 1800). Phytologia: Or, The Philosophy of Agriculture and Gardening. With the Theory of Draining Morasses and with an Improved Construction of the Drill Plough. P. Byrne.
- ^ Ciszak, Marzena; Comparini, Diego; Mazzolai, Barbara; Baluska, Frantisek; Arecchi, F. Tito; Vicsek, Tamás; Mancuso, Stefano (17 January 2012). "Swarming Behavior in Plant Roots". PLOS ONE. 7 (1): e29759. Bibcode:2012PLoSO...729759C. doi:10.1371/journal.pone.0029759. ISSN 1932-6203. PMC 3260168. PMID 22272246.
- ^ Baluška, František; Mancuso, Stefano; Volkmann, Dieter; Barlow, Peter W. (1 July 2010). "Root apex transition zone: a signalling–response nexus in the root". Trends in Plant Science. 15 (7): 402–408. Bibcode:2010TPS....15..402B. doi:10.1016/j.tplants.2010.04.007. PMID 20621671.
- ^ J., Trewavas, A. (2014). Plant behaviour and intelligence. Oxford university press. ISBN 9780199539543. OCLC 961862730.
{{cite book}}
: CS1 maint: multiple names: authors list (link) - ^ Reichenbach H (2001). "Myxobacteria, producers of novel bioactive substances". J Ind Microbiol Biotechnol. 27 (3): 149–56. doi:10.1038/sj.jim.7000025. PMID 11780785. S2CID 34964313.
- ^ Farkas I, Helbing D, Vicsek T (2002). "Mexican waves in an excitable medium" (PDF). Nature. 419 (6903): 131–132. arXiv:cond-mat/0210073. Bibcode:2002Natur.419..131F. doi:10.1038/419131a. PMID 12226653. S2CID 4309609. Archived from the original (PDF) on 10 July 2007.
- ^ Neda Z, Ravasz E, Brechet Y, Vicsek T, Barabasi AL (2002). "Physics of Rhythmic Applause" (PDF). Physical Review E. 61 (6): 6987–6992. arXiv:cond-mat/0006423. Bibcode:2000PhRvE..61.6987N. doi:10.1103/physreve.61.6987. PMID 11088392. S2CID 14135891. Archived from the original (PDF) on 11 June 2011.
- ^ Helbing, D; Keltsch, J; Molnar, P (1997). "Modelling the evolution of human trail systems". Nature. 388 (6637): 47–50. arXiv:cond-mat/9805158. Bibcode:1997Natur.388...47H. doi:10.1038/40353. PMID 9214501. S2CID 4364517.
- ^ "http://psychcentral.com/news/2008/02/15/herd-mentality-explained/1922.html Archived 29 November 2014 at the Wayback Machine". Retrieved on October 31st 2008.
- ^ "Danger in numbers during Haj". The National. 6 September 2009.
- ^ Couzin ID, Krause J (2003). Self-organization and collective behavior in vertebrates (PDF). Advances in the Study of Behavior. Vol. 32. pp. 1–75. doi:10.1016/S0065-3454(03)01001-5. ISBN 978-0-12-004532-7. Archived from the original (PDF) on 13 March 2012. Retrieved 14 April 2011.
- ^ Gabbai, J.M.E. (2005). Complexity and the Aerospace Industry: Understanding Emergence by Relating Structure to Performance using Multi-Agent Systems (Thesis). Manchester: University of Manchester Doctoral Thesis. Archived from the original on 19 December 2014. Retrieved 11 July 2009.
- ^ Livermore R (2008) "A multi-agent system approach to a simulation study comparing the performance of aircraft boarding using pre-assigned seating and free-for-all strategies" Archived 13 August 2011 at the Wayback Machine Open University, Technical report No 2008/25.
- ^ "Planes, Trains and Ant Hills: Computer scientists simulate activity of ants to reduce airline delays" Archived 2010-11-24 at the Wayback Machine Science Daily, 1 April 2008.
- ^ Burd, Martin; N. Aranwela (February 2003). "Head-on encounter rates and walking speed of foragers in leaf-cutting ant traffic". Insectes Sociaux. 50 (1): 3–8. doi:10.1007/s000400300001. S2CID 23876486.
- ^ Ribeiro, Pedro; André Frazão Helene; Gilberto Xavier; Carlos Navas; Fernando Leite Ribeiro (1 April 2009). Dornhaus, Anna (ed.). "Ants can learn to forage on one-way trails". PLOS ONE. 4 (4): e5024. Bibcode:2009PLoSO...4.5024R. doi:10.1371/journal.pone.0005024. PMC 2659768. PMID 19337369.
- ^ John, Alexander; Andreas Schadschneider; Debashish Chowdhury; Katsuhiro Nishinari (March 2008). "Characteristics of ant-inspired traffic flow". Swarm Intelligence. 2 (1): 25–41. arXiv:0903.1434. doi:10.1007/s11721-008-0010-8. S2CID 18350336.
- ^ Are we nearly there yet? Motorists could learn a thing or two from ants The Economist, 10 July 2009.
- ^ Helbing, Dirk; Farkas, Illés; Vicsek, Tamás (2000). "Simulating dynamical features of escape panic". Nature. 407 (6803): 487–490. arXiv:cond-mat/0009448. Bibcode:2000Natur.407..487H. doi:10.1038/35035023. PMID 11028994. S2CID 310346.
- ^ "Swarming the shelves: How shops can exploit people's herd mentality to increase sales?". The Economist. 11 November 2006. p. 90.
- ^ a b Kushleyev, Alex; Mellinger, Daniel; Powers, Caitlin; Kumar, Vijay (2013). "Towards a swarm of agile micro quadrotors". Autonomous Robots. 35 (4): 287–300. doi:10.1007/s10514-013-9349-9. S2CID 18340816.
- ^ "Self-organizing Systems Research Group". Archived from the original on 26 October 2014.
- ^ "Centibots 100-Robot Collaborative Reconnaissance Project". ActivMedia Robotics. Archived from the original on 14 July 2011.
- ^ "Open-source micro-robotic project". Retrieved 28 October 2007.
- ^ "Swarm". iRobot Corporation. Archived from the original on 27 September 2007. Retrieved 28 October 2007.
- ^ Knapp, Louise (21 December 2000). "Look, Up in the Sky: Robofly". Wired. Retrieved 25 September 2008.
- ^ Saska, Martin; Jan, Vakula; Libor, Preucil (2014). Swarms of micro aerial vehicles stabilized under a visual relative localization. IEEE International Conference on Robotics and Automation (ICRA).
- ^ Saska, Martin; Vonasek, Vojtech; Krajnik, Tomas; Preucil, Libor (2014). "Coordination and navigation of heterogeneous MAV–UGV formations localized by a hawk-eye-like approach under a model predictive control scheme" (PDF). International Journal of Robotics Research. 33 (10): 1393–1412. doi:10.1177/0278364914530482. S2CID 1195374.
- ^ Saska, Martin; Vonasek, Vojtech; Krajnik, Tomas; Preucil, Libor (2012). Coordination and Navigation of Heterogeneous UAVs-UGVs Teams Localized by a Hawk-Eye Approach. IEEE/RSJ International Conference on Intelligent Robots and Systems (IROS).
- ^ Ji, Fengtong; Jin, Dongdong; Wang, Ben; Zhang, Li (23 June 2020). "Light-Driven Hovering of a Magnetic Microswarm in Fluid". ACS Nano. 14 (6): 6990–6998. doi:10.1021/acsnano.0c01464. ISSN 1936-0851. PMID 32463226. S2CID 218976382.
- ^ Hughes, Robin (22 February 2007), Barracuda Tornado, retrieved 7 February 2022
- ^ Mou, Fangzhi; Li, Xiaofeng; Xie, Qi; Zhang, Jianhua; Xiong, Kang; Xu, Leilei; Guan, Jianguo (20 December 2019). "Active Micromotor Systems Built from Passive Particles with Biomimetic Predator–Prey Interactions". ACS Nano. 14 (1): 406–414. doi:10.1021/acsnano.9b05996. ISSN 1936-0851. PMID 31860277. S2CID 209435036.
- ^ Yu, Jiangfan; Wang, Ben; Du, Xingzhou; Wang, Qianqian; Zhang, Li (21 August 2018). "Ultra-extensible ribbon-like magnetic microswarm". Nature Communications. 9 (1): 3260. Bibcode:2018NatCo...9.3260Y. doi:10.1038/s41467-018-05749-6. ISSN 2041-1723. PMC 6104072. PMID 30131487.
- ^ Edwards, Sean J.A. (2000). Swarming on the Battlefield: Past, Present, and Future. Rand Monograph MR-1100. Rand Corporation. ISBN 978-0-8330-2779-5.
- ^ U.S. Navy could 'swarm' foes with robot boats, CNN, 13 October 2014.
- ^ "Dive and Discover: Scientific Expedition 10: Antarctica". Retrieved 3 September 2008.
- ^ Crowd modelling: Simulating the behaviour of crowds of people, or swarms of animals, has both frivolous and important uses The Economist, 5 March 2009.
- ^ Fisher, Len (2009) The perfect swarm: the science of complexity in everyday life Page 57. Basic Books. ISBN 978-0-465-01884-0
- ^ Woodford, Riley. "Lemming Suicide Myth Disney Film Faked Bogus Behavior". Archived from the original on 3 January 2010.
- ^ Red-Bellied Piranha Is Really Yellow New York Times, 24 May 2005.
Sources
- Blum C and Merkle D (2008) Swarm intelligence: introduction and applications Springer. ISBN 978-3-540-74088-9.
- Camazine S, Deneubourg JL, Franks NR, Sneyd J, Theraulaz G and Bonabeau E (2003) Self-Organization in Biological Systems Princeton University Press. ISBN 978-0-691-11624-2.
- Fisher L (2009) The perfect swarm: the science of complexity in everyday life Basic Books. ISBN 978-0-465-01884-0.
- Kennedy JF, Kennedy J, Eberhart RC and Shi Y (2001) Swarm intelligence Morgan Kaufmann. ISBN 978-1-55860-595-4.
- Krause, J (2005) Living in Groups Oxford University Press. ISBN 978-0-19-850818-2
- Lim CP, Jain LC and Dehuri S (2009) Innovations in Swarm Intelligence Springer. ISBN 978-3-642-04224-9.
- Miller, Peter (2010) The Smart Swarm: How understanding flocks, schools, and colonies can make us better at communicating, decision making, and getting things done Penguin, ISBN 978-1-58333-390-7
- Nedjah N and Mourelle LdM (2006) Swarm intelligent systems Springer. ISBN 978-3-540-33868-0.
- Sumpter, David JT (2010) Collective Animal Behavior Princeton University Press. ISBN 978-0-691-14843-4.
- Vicsek A, Zafeiris A (2012). "Collective motion". Physics Reports. 517 (3–4): 71–140. arXiv:1010.5017. Bibcode:2012PhR...517...71V. doi:10.1016/j.physrep.2012.03.004. S2CID 119109873.